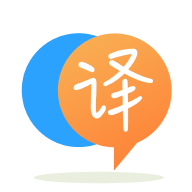
[英]TensorFlow: Compute Hessian matrix (and higher order derivatives)
[英]How to compute all second derivatives (only the diagonal of the Hessian matrix) in Tensorflow?
我有一個損失值/函數,我想計算關於張量f (大小為 n)的所有二階導數。 我設法使用 tf.gradients 兩次,但是當第二次應用它時,它會對第一個輸入的導數求和(請參閱我的代碼中的second_derivatives )。
我也設法檢索了 Hessian 矩陣,但我只想計算它的對角線以避免額外計算。
import tensorflow as tf
import numpy as np
f = tf.Variable(np.array([[1., 2., 0]]).T)
loss = tf.reduce_prod(f ** 2 - 3 * f + 1)
first_derivatives = tf.gradients(loss, f)[0]
second_derivatives = tf.gradients(first_derivatives, f)[0]
hessian = [tf.gradients(first_derivatives[i,0], f)[0][:,0] for i in range(3)]
model = tf.initialize_all_variables()
with tf.Session() as sess:
sess.run(model)
print "\nloss\n", sess.run(loss)
print "\nloss'\n", sess.run(first_derivatives)
print "\nloss''\n", sess.run(second_derivatives)
hessian_value = np.array(map(list, sess.run(hessian)))
print "\nHessian\n", hessian_value
我的想法是tf.gradients(first_derivatives, f[0, 0])[0]可以檢索例如關於 f_0 的二階導數,但似乎 tensorflow 不允許從張量的切片中導出.
tf.gradients([f1,f2,f3],...)
計算f=f1+f2+f3
梯度 此外,關於x[0]
微分是有問題的,因為x[0]
指的是一個新的Slice
節點不是您損失的祖先,因此關於它的導數將為None
。 您可以通過使用pack
將x[0], x[1], ...
粘合到xx
來解決它,並使您的損失取決於xx
而不是x
。 另一種方法是為各個組件使用單獨的變量,在這種情況下,計算 Hessian 看起來像這樣。
def replace_none_with_zero(l):
return [0 if i==None else i for i in l]
tf.reset_default_graph()
x = tf.Variable(1.)
y = tf.Variable(1.)
loss = tf.square(x) + tf.square(y)
grads = tf.gradients([loss], [x, y])
hess0 = replace_none_with_zero(tf.gradients([grads[0]], [x, y]))
hess1 = replace_none_with_zero(tf.gradients([grads[1]], [x, y]))
hessian = tf.pack([tf.pack(hess0), tf.pack(hess1)])
sess = tf.InteractiveSession()
sess.run(tf.initialize_all_variables())
print hessian.eval()
你會看到
[[ 2. 0.]
[ 0. 2.]]
以下函數計算 Tensorflow 2.0 中的二階導數(Hessian 矩陣的對角線):
%tensorflow_version 2.x # Tells Colab to load TF 2.x
import tensorflow as tf
def calc_hessian_diag(f, x):
"""
Calculates the diagonal entries of the Hessian of the function f
(which maps rank-1 tensors to scalars) at coordinates x (rank-1
tensors).
Let k be the number of points in x, and n be the dimensionality of
each point. For each point k, the function returns
(d^2f/dx_1^2, d^2f/dx_2^2, ..., d^2f/dx_n^2) .
Inputs:
f (function): Takes a shape-(k,n) tensor and outputs a
shape-(k,) tensor.
x (tf.Tensor): The points at which to evaluate the Laplacian
of f. Shape = (k,n).
Outputs:
A tensor containing the diagonal entries of the Hessian of f at
points x. Shape = (k,n).
"""
# Use the unstacking and re-stacking trick, which comes
# from https://github.com/xuzhiqin1990/laplacian/
with tf.GradientTape(persistent=True) as g1:
# Turn x into a list of n tensors of shape (k,)
x_unstacked = tf.unstack(x, axis=1)
g1.watch(x_unstacked)
with tf.GradientTape() as g2:
# Re-stack x before passing it into f
x_stacked = tf.stack(x_unstacked, axis=1) # shape = (k,n)
g2.watch(x_stacked)
f_x = f(x_stacked) # shape = (k,)
# Calculate gradient of f with respect to x
df_dx = g2.gradient(f_x, x_stacked) # shape = (k,n)
# Turn df/dx into a list of n tensors of shape (k,)
df_dx_unstacked = tf.unstack(df_dx, axis=1)
# Calculate 2nd derivatives
d2f_dx2 = []
for df_dxi,xi in zip(df_dx_unstacked, x_unstacked):
# Take 2nd derivative of each dimension separately:
# d/dx_i (df/dx_i)
d2f_dx2.append(g1.gradient(df_dxi, xi))
# Stack 2nd derivates
d2f_dx2_stacked = tf.stack(d2f_dx2, axis=1) # shape = (k,n)
return d2f_dx2_stacked
這是一個示例用法,函數f(x) = ln(r)
,其中x
是 3D 坐標, r
是半徑是球坐標:
f = lambda q : tf.math.log(tf.math.reduce_sum(q**2, axis=1))
x = tf.random.uniform((5,3))
d2f_dx2 = calc_hessian_diag(f, x)
print(d2f_dx2)
將看起來像這樣:
tf.Tensor(
[[ 1.415968 1.0215727 -0.25363517]
[-0.67299247 2.4847088 0.70901346]
[ 1.9416015 -1.1799507 1.3937857 ]
[ 1.4748447 0.59702784 -0.52290654]
[ 1.1786096 0.07442689 0.2396735 ]], shape=(5, 3), dtype=float32)
我們可以通過計算拉普拉斯算子(即通過對 Hessian 矩陣的對角線求和)並與我們選擇的函數2 / r^2
的理論答案進行比較來檢查實現的正確性:
print(tf.reduce_sum(d2f_dx2, axis=1)) # Laplacian from summing above results
print(2./tf.math.reduce_sum(x**2, axis=1)) # Analytic expression for Lapalcian
我得到以下信息:
tf.Tensor([2.1839054 2.5207298 2.1554365 1.5489659 1.49271 ], shape=(5,), dtype=float32)
tf.Tensor([2.1839058 2.5207298 2.1554365 1.5489662 1.4927098], shape=(5,), dtype=float32)
他們同意在舍入誤差內。
如果沒有必要使用Tensorflow計算二階導數,您可以:
import sympy as sp
x =sp.symbols('x', real=True)
loss = x**2 - 3*x + 1
f = loss
a = sp.diff(f,x)
b = sp.diff(a)
print ("First derivative of: loss = f ** 2 - 3 * f + 1 respect to f is: ", a,"and the
second derivative is: ",b)
這導致:
First derivative of: loss = f ** 2 - 3 * f + 1 respect to f is: 2*x - 3 and the second derivative is: 2
如果你想計算Hessian,你可以按照這個例子:
import sympy as sp
x,y,z = sp.symbols('x y z', real=True)
f = (x**2)*y*z
Jacobian = np.array([sp.diff(f,x),sp.diff(f,y),sp.diff(f,z)])
Hessian =
np.matrix([[sp.diff(sp.diff(f,x),x),sp.diff(sp.diff(f,y),x),sp.diff(sp.diff(f,z),x)],
[sp.diff(sp.diff(f,x),y),sp.diff(sp.diff(f,y),y),sp.diff(sp.diff(f,z),y)],
[sp.diff(sp.diff(f,x),z),sp.diff(sp.diff(f,z),y),sp.diff(sp.diff(f,z),z)]])
print ("La Jacobian es:", Jacobian)
print ("La Hessian es:\n",Hessian)
這導致:
La Jacobian es: [[2*x*y*z x**2*z x**2*y]]
La Hessian es:
[[2*y*z 2*x*z 2*x*y]
[2*x*z 0 x**2]
[2*x*y x**2 0]]
聲明:本站的技術帖子網頁,遵循CC BY-SA 4.0協議,如果您需要轉載,請注明本站網址或者原文地址。任何問題請咨詢:yoyou2525@163.com.