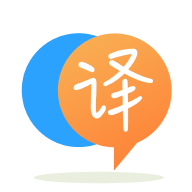
[英]What is the difference between getting score value from kfold,fit,score vs using cross_val_score?
[英]Getting probabilities from cross_val_score
我有以下使用嵌套交叉驗證的python機器學習管道:
from sklearn.model_selection import StratifiedShuffleSplit
from sklearn.svm import SVC
sss_outer = StratifiedShuffleSplit(n_splits=5, test_size=0.4, random_state=15)
sss_inner = StratifiedShuffleSplit(n_splits=3, test_size=0.2, random_state=16)
pipe_svm = Pipeline([('scl', StandardScaler()), ('clf', SVC(kernel="linear"))])
parameters = {'clf__C': logspace(-4, 1, 50)}
grid_search = GridSearchCV(estimator=pipe_svm, param_grid=parameters, verbose=1, scoring='roc_auc', cv=sss_inner)
cross_val_score(grid_search, X, y, cv=sss_outer)
現在,我想從cross_val_score中獲取概率,以便可以計算AUC並繪制ROC和精度/召回曲線。 如何才能做到這一點?
您可以使用sklearn.metrics.roc_curve函數來計算模型的ROC得分
這是使用SVM分類器的示例代碼片段:
from sklearn.model_selection import StratifiedShuffleSplit
from sklearn.svm import SVC
from sklearn.metrics import roc_curve, auc
X = {
"train": [...],
"text": [...]
}
Y = {
"train": [...],
"text": [...]
}
sss_outer = StratifiedShuffleSplit(n_splits=5, test_size=0.4, random_state=15)
sss_inner = StratifiedShuffleSplit(n_splits=3, test_size=0.2, random_state=16)
pipe_svm = Pipeline([('scl', StandardScaler()), ('clf', SVC(kernel="linear"))])
parameters = {'clf__C': logspace(-4, 1, 50)}
grid_search = GridSearchCV(estimator=pipe_svm, param_grid=parameters, verbose=1, scoring='roc_auc', cv=sss_inner)
probas_ = grid_search.fit(X[train], y[train]).predict_proba(X[test])
fpr, tpr, thresholds = roc_curve(y[test], probas_[:, 1])
roc_auc = auc(fpr, tpr)
您還可以訪問sklearn示例: 具有交叉驗證的接收器工作特性(ROC),以了解更多詳細信息。
希望能幫助到你。
## 3. set up cross validation method
inner_cv = RepeatedStratifiedKFold(n_splits=10, n_repeats=5)
outer_cv = RepeatedStratifiedKFold(n_splits=10, n_repeats=5)
## 4. set up inner cross validation parameter tuning, can use this to get AUC
log.model = GridSearchCV(estimator=log, param_grid=log_hyper, cv=inner_cv, scoring='roc_auc')
## 5. ordinary nested cross validation without probabilities
log_scores = cross_val_score(log.model, X, Y, scoring='roc_auc', cv=outer_cv)
print("AUC: %0.2f (+/- %0.2f)" % (log_scores.mean(), log_scores.std() * 2))
## 6. this is to get the probabilities from nested cross validation
log_scores2 = cross_val_predict(log.model, X, Y, cv=outer_cv,method='predict_proba')
fpr, tpr, thresholds = roc_curve(Y, log_scores2[:, 1])
roc_auc = auc(fpr, tpr)
您可以使用cross_val_predict函數進行嵌套的交叉驗證,並獲得ROC曲線的概率。 據我所知,GridSearchCV不允許像cross_val_predict那樣提取交叉驗證的概率。
我假設cross_val_predict的值是所有迭代的平均概率。 您無法從cross_val_score獲得交叉驗證的概率。
如果不確定方法是否適合,請嘗試對隨機數據運行陰性對照。 這是一個好習慣。
聲明:本站的技術帖子網頁,遵循CC BY-SA 4.0協議,如果您需要轉載,請注明本站網址或者原文地址。任何問題請咨詢:yoyou2525@163.com.