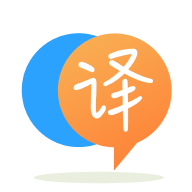
[英]Python Pandas DataFrame User Defined Function Transformations
[英]Vectorising pandas dataframe apply function for user defined function in python
我要計算指定日期的一周中的某周。 對於每月的第幾周,我目前使用用戶定義的函數。
輸入數據幀:
輸出數據幀:
這是我嘗試過的:
from math import ceil
def week_of_month(dt):
"""
Returns the week of the month for the specified date.
"""
first_day = dt.replace(day=1)
dom = dt.day
adjusted_dom = dom + first_day.weekday()
return int(ceil(adjusted_dom/7.0))
在這之后,
import pandas as pd
df = pd.read_csv("input_dataframe.csv")
df.date = pd.to_datetime(df.date)
df['year_of_date'] = df.date.dt.year
df['month_of_date'] = df.date.dt.month
df['day_of_date'] = df.date.dt.day
wom = pd.Series()
# worker function for creating week of month series
def convert_date(t):
global wom
wom = wom.append(pd.Series(week_of_month(datetime.datetime(t[0],t[1],t[2]))), ignore_index = True)
# calling worker function for each row of dataframe
_ = df[['year_of_date','month_of_date','day_of_date']].apply(convert_date, axis = 1)
# adding new computed column to dataframe
df['week_of_month'] = wom
# here this updated dataframe should look like Output data frame.
這是針對使用給定函數計算的數據行的每一行進行的。 隨着數據幀增加到更多行,它使計算速度變慢。 因為目前我有超過1000萬行。
我正在尋找一種更快的方法。 我可以對此代碼進行哪些更改以在所有行上向量化此操作?
提前致謝。
編輯:閱讀答案后對我有用的是下面的代碼,
first_day_of_month = pd.to_datetime(df.date.values.astype('datetime64[M]'))
df['week_of_month'] = np.ceil((df.date.dt.day + first_day_of_month.weekday) / 7.0).astype(int)
可以對week_of_month
方法進行向量化。 不執行到datetime對象的轉換,而只使用pandas方法可能是有益的。
first_day_of_month = df.date.to_period("M").to_timestamp()
df["week_of_month"] = np.ceil((data.day + first_day_of_month.weekday) / 7.0).astype(int)
甚至不用花很多時間編寫代碼,也不會提到X / Y問題,等等:
嘗試獲取唯一日期的列表,我敢肯定,在1000萬行中,您有多個是重復的。
腳步:
聲明:本站的技術帖子網頁,遵循CC BY-SA 4.0協議,如果您需要轉載,請注明本站網址或者原文地址。任何問題請咨詢:yoyou2525@163.com.