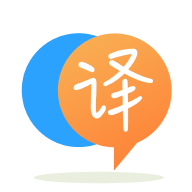
[英]Getting a prediction from a loaded tensor flow classification model, .pd file
[英]Get a prediction from Tensor Flow Model
我想從訓練有素的張量流模型中獲得預測。 以下是我用於訓練模型的代碼。
def train_model(self, train, test, learning_rate=0.0001, num_epochs=16, minibatch_size=32, print_cost=True, graph_filename='costs'):
# Ensure that model can be rerun without overwriting tf variables
ops.reset_default_graph()
# For reproducibility
tf.set_random_seed(42)
seed = 42
# Get input and output shapes
(n_x, m) = train.images.T.shape
n_y = train.labels.T.shape[0]
costs = []
# Create placeholders of shape (n_x, n_y)
X, Y = self.create_placeholders(n_x, n_y)
# Initialize parameters
parameters = self.initialize_parameters()
# Forward propagation
Z3 = self.forward_propagation(X, parameters)
# Cost function
cost = self.compute_cost(Z3, Y)
# Backpropagation (using Adam optimizer)
optimizer = tf.train.AdamOptimizer(learning_rate).minimize(cost)
# Initialize variables
init = tf.global_variables_initializer()
# Start session to compute Tensorflow graph
with tf.Session() as sess:
# Run initialization
sess.run(init)
# Training loop
for epoch in range(num_epochs):
epoch_cost = 0.
num_minibatches = int(m / minibatch_size)
seed = seed + 1
for i in range(num_minibatches):
# Get next batch of training data and labels
minibatch_X, minibatch_Y = train.next_batch(minibatch_size)
# Execute optimizer and cost function
_, minibatch_cost = sess.run([optimizer, cost], feed_dict={X: minibatch_X.T, Y: minibatch_Y.T})
# Update epoch cost
epoch_cost += minibatch_cost / num_minibatches
# Print the cost every epoch
if print_cost == True:
print("Cost after epoch {epoch_num}: {cost}".format(epoch_num=epoch, cost=epoch_cost))
costs.append(epoch_cost)
# Plot costs
plt.figure(figsize=(16,5))
plt.plot(np.squeeze(costs), color='#2A688B')
plt.xlim(0, num_epochs-1)
plt.ylabel("cost")
plt.xlabel("iterations")
plt.title("learning rate = {rate}".format(rate=learning_rate))
plt.savefig(graph_filename, dpi=300)
plt.show()
# Save parameters
parameters = sess.run(parameters)
print("Parameters have been trained!")
# Calculate correct predictions
correct_prediction = tf.equal(tf.argmax(Z3), tf.argmax(Y))
# Calculate accuracy on test set
accuracy = tf.reduce_mean(tf.cast(correct_prediction, "float"))
print ("Train Accuracy:", accuracy.eval({X: train.images.T, Y: train.labels.T}))
print ("Test Accuracy:", accuracy.eval({X: test.images.T, Y: test.labels.T}))
return parameters
訓練模型后,我想從模型中提取預測。 所以我加
print(sess.run(accuracy, feed_dict={X: test.images.T}))
但是運行上面的代碼后,我看到以下錯誤:
InvalidArgumentError:您必須使用dtype float和形狀[10 ,?] [[{{{node Y}} = Placeholderdtype = DT_FLOAT,shape = [10 ,?],_device =“ / job:”輸入占位符張量'Y'的值:本地主機/副本:0 /任務:0 /設備:CPU:0“]]
歡迎任何幫助。
張量accuracy
是張量correct_prediction
的函數,而張量correct_prediction
又是(除其他之外) Y
的函數。
因此,您被正確告知您也應該為該占位符提供值。
我假設Y
保留了您的標簽,因此從直觀上來說,您的feed_dict也將包含正確的Y
值。
希望能有所幫助。
祝好運!
聲明:本站的技術帖子網頁,遵循CC BY-SA 4.0協議,如果您需要轉載,請注明本站網址或者原文地址。任何問題請咨詢:yoyou2525@163.com.