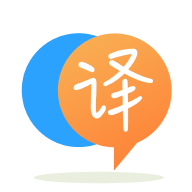
[英]ValueError: shapes (100,1) and (2,1) not aligned: 1 (dim 1) != 2 (dim 0)
[英]Linear Regressor unable to predict a set of values; Error: ValueError: shapes (100,1) and (2,1) not aligned: 1 (dim 1) != 2 (dim 0)
我有 2 個 numpy 數組:
x= np.linspace(1,10,100) + np.random.randn(n)/5
y = np.sin(x)+x/6 + np.random.randn(n)/10
我想使用這些數據集訓練線性回歸器。 為了比較復雜性和泛化之間的關系,我對一組 4 度(1, 3, 6, 9)
使用了 h 多項式特征預處理。 擬合模型后,我想測試一個數組x = np.linspace(1, 10, 100)
經過多次嘗試,我發現 x 和 y 數組需要重新整形,我就這樣做了。 但是,當我創建要預測的新 x 數據集時,它會抱怨維度未對齊。 估計器正在處理原始 x 數組的測試拆分。
下面是我的代碼
import numpy as np
import pandas as pd
from sklearn.model_selection import train_test_split
np.random.seed(0)
n = 100
x = np.linspace(0,10,n) + np.random.randn(n)/5
y = np.sin(x)+x/6 + np.random.randn(n)/10
X_train, X_test, y_train, y_test = train_test_split(x, y, random_state=0)
def fn_one():
from sklearn.linear_model import LinearRegression
from sklearn.preprocessing import PolynomialFeatures
x_predict = np.linspace(0,10,100)
x_predict = x_predict.reshape(-1, 1)
degrees = [1, 3, 6, 9]
predictions = []
for i, deg in enumerate(degrees):
linReg = LinearRegression()
pf = PolynomialFeatures(degree=deg)
xt = x.reshape(-1, 1)
yt = y.reshape(-1, 1)
X_transformed = pf.fit_transform(xt)
X_train_transformed, X_test_transformed, y_train_temp, y_test_temp = train_test_split(X_transformed, yt, random_state=0)
linReg.fit(X_train_transformed, y_train_temp)
predictions.append(linReg.predict(x_predict))
np.array(predictions)
return predictions
不同數組的形狀(@循環中的度數 3)
x_predict = (100, 1)
xt = 100, 1
yt = 100, 1
X_train_transformed = 75, 4
y_train_temp = 75, 1
X_test_transformed = 25, 4
y_train_temp = 25, 1
X_test_transformed = 4, 25, 1 的預測
x_predict 的預測 = 不工作:
錯誤 = ValueError:形狀 (100,1) 和 (2,1) 未對齊:1 (dim 1) != 2 (dim 0)
你忘了轉換你的x_predict
。 我已在下面更新了您的代碼:
import numpy as np
import pandas as pd
from sklearn.model_selection import train_test_split
np.random.seed(0)
n = 100
x = np.linspace(0,10,n) + np.random.randn(n)/5
y = np.sin(x)+x/6 + np.random.randn(n)/10
X_train, X_test, y_train, y_test = train_test_split(x, y, random_state=0)
def fn_one():
from sklearn.linear_model import LinearRegression
from sklearn.preprocessing import PolynomialFeatures
x_predict = np.linspace(0,10,100)
x_predict = x_predict.reshape(-1, 1)
degrees = [1, 3, 6, 9]
predictions = []
for i, deg in enumerate(degrees):
linReg = LinearRegression()
pf = PolynomialFeatures(degree=deg)
xt = x.reshape(-1, 1)
yt = y.reshape(-1, 1)
X_transformed = pf.fit_transform(xt)
X_train_transformed, X_test_transformed, y_train_temp, y_test_temp = train_test_split(X_transformed, yt, random_state=0)
linReg.fit(X_train_transformed, y_train_temp)
x_predict_transformed = pf.fit_transform(x_predict)
predictions.append(linReg.predict(x_predict_transformed))
np.array(predictions)
return predictions
現在,當您調用fn_one()
您將獲得預測。
希望這可以幫助!
聲明:本站的技術帖子網頁,遵循CC BY-SA 4.0協議,如果您需要轉載,請注明本站網址或者原文地址。任何問題請咨詢:yoyou2525@163.com.