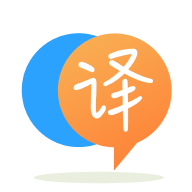
[英]TF2.0 image generator not working using Keras ImageDataGenerator
[英]How to generate CNN heatmaps using built-in Keras in TF2.0 (tf.keras)
我曾經為我的卷積神經網絡生成熱圖,基於 TensorFlow 1 之上的獨立 Keras 庫。但是,在我切換到 TF2.0 和內置tf.keras
執行之后效果很好) 我不能再使用舊的熱圖生成代碼了。
因此,我為 TF2.0 重新編寫了部分代碼,結果如下:
from tensorflow.keras.applications.vgg16 import preprocess_input
from tensorflow.keras.preprocessing.image import load_img
from tensorflow.keras.models import load_model
from tensorflow.keras import preprocessing
from tensorflow.keras import backend as K
from tensorflow.keras import models
import matplotlib.pyplot as plt
import tensorflow as tf
import numpy as np
image_size = 150
image_path = "/tmp/images/test-image.jpg"
model_path = "/tmp/models/prototype/basic_vgg16.h5"
# Load pre-trained Keras model and the image to classify
model = load_model(model_path) # VGG16 CNN with custom classifier head
image = load_img(image_path, target_size=(image_size, image_size))
img_tensor = preprocessing.image.img_to_array(image)
img_tensor = np.expand_dims(img_tensor, axis=0)
img_tensor = preprocess_input(img_tensor)
input_layer = model.get_layer("model_input")
conv_layer = model.get_layer("block5_conv3")
heatmap_model = models.Model([model.inputs], [conv_layer.output, model.output])
# Get gradient of the winner class w.r.t. the output of the (last) conv. layer
with tf.GradientTape() as gtape:
conv_output, predictions = heatmap_model(img_tensor)
loss = predictions[:, np.argmax(predictions[0])]
grads = gtape.gradient(loss, conv_output)
pooled_grads = K.mean(grads, axis=(0, 1, 2))
# Get values of pooled grads and model conv. layer output as Numpy arrays
iterate = K.function([model.inputs], [pooled_grads, conv_layer.output[0]])
pooled_grads_value, conv_layer_output_value = iterate([img_tensor])
# Multiply each channel in the feature-map array by "how important it is"
for i in range(pooled_grads_value.shape[0]):
conv_layer_output_value[:, :, i] *= pooled_grads_value[i]
# Channel-wise mean of resulting feature-map is the heatmap of class activation
heatmap = np.mean(conv_layer_output_value, axis=-1)
heatmap = np.maximum(heatmap, 0)
max_heat = np.max(heatmap)
if max_heat == 0:
max_heat = 1e-10
heatmap /= max_heat
# Render heatmap via pyplot
plt.matshow(heatmap)
plt.show()
但現在以下行:
iterate = K.function([model.inputs], [pooled_grads, conv_layer.output[0]])
導致此錯誤消息:
AttributeError: Tensor.op is meaningless when eager execution is enabled.
我一直使用 Keras 並沒有直接使用 TF,所以我在這里有點迷失了。
有什么想法可能是這里的問題嗎?
PS:如果你想c&p這段代碼,你可以像這樣創建基於VGG16的model:
# Create Keras model from pre-trained VGG16 and custom classifier
input_layer = layers.Input(shape=(image_size, image_size, 3), name="model_input")
vgg16_model = VGG16(weights="imagenet", include_top=False, input_tensor=input_layer)
model_head = vgg16_model.output
model_head = layers.Flatten(name="model_head_flatten")(model_head)
model_head = layers.Dense(256, activation="relu")(model_head)
model_head = layers.Dense(3, activation="softmax")(model_head)
model = models.Model(inputs=input_layer, outputs=model_head)
model.compile(loss="categorical_crossentropy", optimizer=optimizers.Adam(), metrics=["accuracy"])
在GradientTape
循環結束時, conv_output
和grads
已經保存了該值。 迭代 function 不再需要計算值。
下面的工作示例:
from tensorflow.keras.applications.vgg16 import preprocess_input
from tensorflow.keras.preprocessing.image import load_img
from tensorflow.keras.models import load_model
from tensorflow.keras import preprocessing
from tensorflow.keras import backend as K
from tensorflow.keras import models
import tensorflow as tf
import numpy as np
image_size = 224
# Load pre-trained Keras model and the image to classify
model = tf.keras.applications.vgg16.VGG16()
image = np.random.random((image_size, image_size, 3))
img_tensor = preprocessing.image.img_to_array(image)
img_tensor = np.expand_dims(img_tensor, axis=0)
img_tensor = preprocess_input(img_tensor)
conv_layer = model.get_layer("block5_conv3")
heatmap_model = models.Model([model.inputs], [conv_layer.output, model.output])
# Get gradient of the winner class w.r.t. the output of the (last) conv. layer
with tf.GradientTape() as gtape:
conv_output, predictions = heatmap_model(img_tensor)
loss = predictions[:, np.argmax(predictions[0])]
grads = gtape.gradient(loss, conv_output)
pooled_grads = K.mean(grads, axis=(0, 1, 2))
heatmap = tf.reduce_mean(tf.multiply(pooled_grads, conv_output), axis=-1)
heatmap = np.maximum(heatmap, 0)
max_heat = np.max(heatmap)
if max_heat == 0:
max_heat = 1e-10
heatmap /= max_heat
print(heatmap.shape)
聲明:本站的技術帖子網頁,遵循CC BY-SA 4.0協議,如果您需要轉載,請注明本站網址或者原文地址。任何問題請咨詢:yoyou2525@163.com.