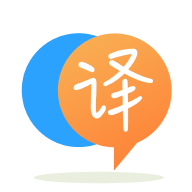
[英]Find number of consecutively increasing/decreasing values in a pandas column (and fill another col with it) in an optimized way
[英]How to find the highest count of sequential (numbers|increasing|decreasing) in pandas DataFrame column of values
如何在同一列中找到連續出現的最高計數,例如相同的數字、遞增的值或遞減的值。
所以給出類似的東西:
h_diff l_diff monotonic
timestamp
2000-01-18 NaN NaN NaN
2000-01-19 2.75 2.93 1.0
2000-01-20 12.75 10.13 1.0
2000-01-21 -7.25 -3.31 0.0
2000-01-24 -1.50 -5.07 0.0
2000-01-25 0.37 -2.75 1.0
2000-01-26 1.07 7.38 1.0
2000-01-27 -1.19 -2.75 0.0
2000-01-28 -2.13 -6.38 0.0
2000-01-31 -7.00 -6.12 0.0
h_diff 中正值的單調性最高值為 2,負值的單調性最高值為 3。 l_diff 相同。 因此,如果滾動為 10 或 n,我將如何找到最高單調計數,同時仍然能夠動態更改窗口大小?
這給了我單調列的 1.0 值: lambda x: np.all(np.diff(x) > 0) 和 lambda x: np.count_nonzero(np.diff(x) > 0) 將計算總計數1.0 用於窗口,但我試圖找到的是一系列給定窗口中運行時間最長的。
我希望的是這樣的:
h_diff l_diff monotonic
timestamp
2000-01-18 NaN NaN NaN
2000-01-19 2.75 2.93 1.0
2000-01-20 12.75 10.13 2.0
2000-01-21 -7.25 -3.31 0.0
2000-01-24 -1.50 -5.07 0.0
2000-01-25 0.37 -2.75 1.0
2000-01-26 1.07 7.38 2.0
2000-01-27 1.19 -2.75 3.0
2000-01-28 2.13 -6.38 4.0
2000-01-31 -7.00 -6.12 0.0
下面的代碼應該可以找到連續出現的正數或負數的技巧。 下面的代碼用於列h_diff
df1[df1.h_diff.gt(0)].index.to_series().diff().ne(1).cumsum().value_counts().max() #sequential occurrences greater than 0
df1[df1.h_diff.lt(0)].index.to_series().diff().ne(1).cumsum().value_counts().max() #sequential occurrences less than 0
使用GroupBy.cumcount
+ Series.where
。
初始數據幀
h_diff l_diff
timestamp
2000-01-18 NaN NaN
2000-01-19 2.75 2.93
2000-01-20 12.75 10.13
2000-01-21 -7.25 -3.31
2000-01-24 -1.50 -5.07
2000-01-25 0.37 -2.75
2000-01-26 1.07 7.38
2000-01-27 1.19 -2.75
2000-01-28 2.13 -6.38
2000-01-31 -7.00 -6.12
h = df['h_diff'].gt(0)
#h = np.sign(df['h_diff'])
df['monotonic_h']=h.groupby(h.ne(h.shift()).cumsum()).cumcount().add(1).where(h,0)
print(df)
h_diff l_diff monotonic_h
timestamp
2000-01-18 NaN NaN 0
2000-01-19 2.75 2.93 1
2000-01-20 12.75 10.13 2
2000-01-21 -7.25 -3.31 0
2000-01-24 -1.50 -5.07 0
2000-01-25 0.37 -2.75 1
2000-01-26 1.07 7.38 2
2000-01-27 1.19 -2.75 3
2000-01-28 2.13 -6.38 4
2000-01-31 -7.00 -6.12 0
df['monotonic_h'].max()
#4
細節
h.ne(h.shift()).cumsum()
timestamp
2000-01-18 1
2000-01-19 2
2000-01-20 2
2000-01-21 3
2000-01-24 3
2000-01-25 4
2000-01-26 4
2000-01-27 4
2000-01-28 4
2000-01-31 5
Name: h_diff, dtype: int64
更新
df = df.join( h.groupby(h.ne(h.shift()).cumsum()).cumcount().add(1)
.to_frame('values')
.assign(monotic = np.where(h,'monotic_h_greater_0',
'monotic_h_not_greater_0'),
index = lambda x: x.index)
.where(df['h_diff'].notna())
.pivot_table(columns = 'monotic',
index = 'index',
values = 'values',
fill_value=0) )
print(df)
h_diff l_diff monotic_h_greater_0 monotic_h_not_greater_0
timestamp
2000-01-18 NaN NaN NaN NaN
2000-01-19 2.75 2.93 1.0 0.0
2000-01-20 12.75 10.13 2.0 0.0
2000-01-21 -7.25 -3.31 0.0 1.0
2000-01-24 -1.50 -5.07 0.0 2.0
2000-01-25 0.37 -2.75 1.0 0.0
2000-01-26 1.07 7.38 2.0 0.0
2000-01-27 1.19 -2.75 3.0 0.0
2000-01-28 2.13 -6.38 4.0 0.0
2000-01-31 -7.00 -6.12 0.0 1.0
聲明:本站的技術帖子網頁,遵循CC BY-SA 4.0協議,如果您需要轉載,請注明本站網址或者原文地址。任何問題請咨詢:yoyou2525@163.com.