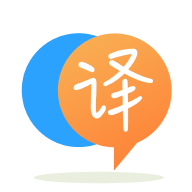
[英]Tensorflow error in Colab - ValueError: Shapes (None, 1) and (None, 10) are incompatible
[英]ValueError: Shapes (None, 50) and (None, 1) are incompatible in Tensorflow and Colab
我正在使用 LSTM 訓練 Tensorflow model 以進行預測性維護。 對於每個實例,我創建一個矩陣 (50,4),其中 50 是歷史序列的長度,4 是每個記錄的特征數,因此為了訓練 model,我使用例如 (55048, 50, 4) 張量和a (55048, 1) 作為標簽。 當我在計算機上使用 Jupyter 進行訓練時,它可以工作(非常慢,但可以),但在 Colab 上我收到此錯誤:
Training data shape is (55048, 50, 4)
Labels shape is (55048, 1)
WARNING:tensorflow:Layer lstm will not use cuDNN kernel since it doesn't meet the cuDNN kernel criteria. It will use generic GPU kernel as fallback when running on GPU
Model: "sequential"
_________________________________________________________________
Layer (type) Output Shape Param #
=================================================================
lstm (LSTM) (None, 50, 100) 42000
_________________________________________________________________
dense (Dense) (None, 50, 1) 101
=================================================================
Total params: 42,101
Trainable params: 42,101
Non-trainable params: 0
_________________________________________________________________
Epoch 1/50
WARNING:tensorflow:From /usr/local/lib/python3.6/dist-packages/tensorflow/python/ops/resource_variable_ops.py:1817: calling BaseResourceVariable.__init__ (from tensorflow.python.ops.resource_variable_ops) with constraint is deprecated and will be removed in a future version.
Instructions for updating:
If using Keras pass *_constraint arguments to layers.
ValueError: in user code:
/usr/local/lib/python3.6/dist-packages/tensorflow/python/keras/engine/training.py:571 train_function *
outputs = self.distribute_strategy.run(
/usr/local/lib/python3.6/dist-packages/tensorflow/python/distribute/distribute_lib.py:951 run **
return self._extended.call_for_each_replica(fn, args=args, kwargs=kwargs)
/usr/local/lib/python3.6/dist-packages/tensorflow/python/distribute/distribute_lib.py:2290 call_for_each_replica
return self._call_for_each_replica(fn, args, kwargs)
/usr/local/lib/python3.6/dist-packages/tensorflow/python/distribute/distribute_lib.py:2649 _call_for_each_replica
return fn(*args, **kwargs)
/usr/local/lib/python3.6/dist-packages/tensorflow/python/keras/engine/training.py:543 train_step **
self.compiled_metrics.update_state(y, y_pred, sample_weight)
/usr/local/lib/python3.6/dist-packages/tensorflow/python/keras/engine/compile_utils.py:406 update_state
metric_obj.update_state(y_t, y_p)
/usr/local/lib/python3.6/dist-packages/tensorflow/python/keras/utils/metrics_utils.py:90 decorated
update_op = update_state_fn(*args, **kwargs)
/usr/local/lib/python3.6/dist-packages/tensorflow/python/keras/metrics.py:2083 update_state
label_weights=label_weights)
/usr/local/lib/python3.6/dist-packages/tensorflow/python/keras/utils/metrics_utils.py:351 update_confusion_matrix_variables
y_pred.shape.assert_is_compatible_with(y_true.shape)
/usr/local/lib/python3.6/dist-packages/tensorflow/python/framework/tensor_shape.py:1117 assert_is_compatible_with
raise ValueError("Shapes %s and %s are incompatible" % (self, other))
ValueError: Shapes (None, 50) and (None, 1) are incompatible
我與您分享一些代碼。 我知道它很長:
def build_lstm(train_data, train_labels, structure=(100,), epochs=50, activation_fun="relu", dropout_rate=0.1,
loss_function="binary_crossentropy", optimizer="adagrad", val_split=0.2, seq_length=50):
#n_features = len(train_data.columns)
print("Train data is\n",train_data)
acceptable_ids = [idx for idx in train_data['id'].unique() if train_data[train_data['id']==idx].shape[0]>seq_length]
seq_gen = [list(gen_sequence(train_data[train_data['id']==idx], seq_length)) for idx in acceptable_ids]
print("Seq gen is\n")
print(np.array(seq_gen).shape)
seq_array = np.concatenate(seq_gen,0).astype(np.float32)
print("Training data shape is", seq_array.shape)
#train_labels = np.asarray(train_labels).astype('float32').reshape((-1,1))
label_gen = [gen_labels(train_labels[train_labels['id']==idx], seq_length) for idx in acceptable_ids]
label_array = np.concatenate(label_gen).astype(np.float32)
print("Labels shape is", label_array.shape)
first_layer=True
model = tf.keras.Sequential()
for layer_nodes in structure:
if first_layer:
model.add(LSTM(layer_nodes, activation=activation_fun, input_shape=(seq_length,train_data.shape[1]-1),
dropout=dropout_rate, return_sequences=True))
first_layer=False
else:
model.add(LSTM(layer_nodes, activation=activation_fun,
dropout=dropout_rate, return_sequences=False))
model.add(Dense(1, activation='sigmoid'))
model.summary()
model.compile(loss=loss_function,
optimizer=optimizer,
metrics=['AUC','accuracy'])
history = model.fit(seq_array,label_array, epochs=epochs, shuffle=True, validation_split=val_split, callbacks=[earlystop_callback])
return model
def gen_sequence(id_df, seq_length):
""" Only sequences that meet the window-length are considered, no padding is used. This means for testing
we need to drop those which are below the window-length. An alternative would be to pad sequences so that
we can use shorter ones """
# for one id I put all the rows in a single matrix
data_matrix = id_df.drop("id",1).values
num_elements = data_matrix.shape[0]
# Iterate over two lists in parallel.
# For example id1 have 192 rows and sequence_length is equal to 50
# so zip iterate over two following list of numbers (0,112),(50,192)
# 0 50 -> from row 0 to row 50
# 1 51 -> from row 1 to row 51
# 2 52 -> from row 2 to row 52
# ...
# 111 191 -> from row 111 to 191
for start, stop in zip(range(0, num_elements-seq_length), range(seq_length, num_elements)):
#print(data_matrix[start:stop, :],"\n")
yield data_matrix[start:stop, :]
def gen_labels(id_df, seq_length):
data_array = id_df.drop("id",1).values
num_elements = data_array.shape[0]
return data_array[seq_length:num_elements, :]
...
for comb_hyp in hyp_combinations:
for id_validation in training_folds_2:
print(id_validation)
## SEPARATE TRAINING SET AND VALIDATION SET
X_val = X[X.id.isin(id_validation)].copy()
X_train = X[~X.id.isin(id_validation)].copy()
y_val = y[y.id.isin(id_validation)].copy()
y_train = y[~y.id.isin(id_validation)].copy()
## TRAIN THE CLASSIFIER
clf = build_lstm(train_data=X_train, train_labels=y_train, structure=comb_hyp[2], epochs=EPOCHS, activation_fun=comb_hyp[0], optimizer=SOLVER, seq_length=SEQ_LENGTH)
...
為什么它在 Jupyter 中有效,而在 Colab 中無效? 感謝您的關注。
就我而言,我卸載tensorflow
然后安裝了tensorflow-gpu
問題就解決了
我已經將運行時設置為 GPU。 如果我將最后一層不是具有一個節點的密集層(用於二進制分類)而是具有一個節點的 LSTM 層作為最后一層,它就可以工作。 也許是因為 LSTM 和 Dense 不應該混用。 謝謝您的回復。
聲明:本站的技術帖子網頁,遵循CC BY-SA 4.0協議,如果您需要轉載,請注明本站網址或者原文地址。任何問題請咨詢:yoyou2525@163.com.