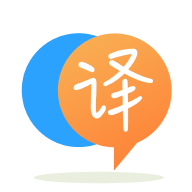
[英]input/output/recurrent dropout layers in BiLSTM_Classifier and how they affect the model and prediction
[英]Not using recurrent_dropout in colab crashing model?
我正在嘗試訓練一個簡單的 tensorflow model 來檢測推文的情緒。 arrays 的數據類型和大小是一致的,並且當經常性丟棄設置為某個浮點值時,model 訓練得很好。 但是,這會禁用 cuDNN,我真的很想加快速度(不是我們所有人),但是每當我刪除經常性 dropout 參數時,model 訓練將在第一個時期結束之前崩潰。
下面是相關代碼,我省略了導入,並加載了 csv 文件。 相關代碼之后是最終輸入尺寸和錯誤代碼。 此外,我已經弄清楚為什么 colab 似乎在削減訓練數據。 Colab 會在拆分成批次后顯示序列數,因此默認批次大小為 32,我們得到 859 個序列。 不使用經常性 dropout 時的崩潰問題仍然是一個問題。 旁注,這段代碼是一個非常粗略的草稿,數據清理都在同一個筆記本中完成,因此缺乏典型的格式。
def remove_case(X):
removed_case = []
X = X.copy()
for text in X:
text = str(text).lower()
removed_case.append(text)
X = removed_case
return X
def remove_hyperlinks(X):
removed_hyperlinks = []
X = X.copy()
for text in X:
text = str(text)
text = re.sub(r'http\S+', '', text)
text = re.sub(r'https\S+', '', text)
text = re.sub(r'www\S+', '', text)
removed_hyperlinks.append(text)
X = removed_hyperlinks
return X
def remove_punctuation(X):
removed_punc = []
X = X.copy()
for text in X:
text = str(text)
text = "".join([char for char in text if char not in punctuation])
removed_punc.append(text)
X = removed_punc
return X
def split_text(X):
split_tweets = []
X = X.copy()
for text in X:
text = str(text).split()
split_tweets.append(text)
X = split_tweets
return X
def map_sentiment(X, l, m, n):
keys = ['negative', 'neutral', 'positive']
values = [l, m, n]
dictionary = dict(zip(keys, values))
X = X.copy()
X = X.map(dictionary)
return X
# # def sentiment_to_onehot(X):
# sentiment_foofs = []
# X = X.copy()
# for integer in X:
# if integer == "negative": # Negative
# integer = [1, 0, 0]
# elif integer == "neutral": # Neutral
# integer = [0, 1, 0]
# elif integer == "positive": # Positive
# integer = [0, 0, 1]
# else:
# break
# sentiment_foofs.append(integer)
# X = sentiment_foofs
# return X
train_no_punc_lowercase = train.copy()
train_no_punc_lowercase['text'] = remove_case(train_no_punc_lowercase['text'])
train_no_punc_lowercase['text'] = remove_hyperlinks(train_no_punc_lowercase['text'])
train_no_punc_lowercase['text'] = remove_punctuation(train_no_punc_lowercase['text'])
train_no_punc_lowercase['sentiment'] = map_sentiment(train_no_punc_lowercase['sentiment'], 0, 1, 2)
train_no_punc_lowercase.head()
test_no_punc_lowercase = test.copy()
test_no_punc_lowercase['text'] = remove_case(test_no_punc_lowercase['text'])
test_no_punc_lowercase['text'] = remove_hyperlinks(test_no_punc_lowercase['text'])
test_no_punc_lowercase['text'] = remove_punctuation(test_no_punc_lowercase['text'])
test_no_punc_lowercase['sentiment'] = map_sentiment(test_no_punc_lowercase['sentiment'], 0, 1, 2)
features = train.columns.tolist()
features.remove('textID') # all unique, high cardinality feature
features.remove('selected_text') # target
target = 'selected_text'
X_train_no_punc_lowercase = train_no_punc_lowercase[features]
y_train_no_punc_lowercase = train_no_punc_lowercase[target]
X_test_no_punc_lowercase = test_no_punc_lowercase[features]
def stemming_column(df_column):
ps = PorterStemmer()
stemmed_word_list = []
for i, string in enumerate(df_column):
tokens = word_tokenize(string)
new_string = ""
for j, words in enumerate(tokens):
new_string = new_string + ps.stem(words) + " "
stemmed_word_list.append(new_string)
return stemmed_word_list
def create_lookup_table(list1, list2):
main_list = []
lookup_dict = {}
i = 1 # used to create a value in the dictionary
main_list.append(list1)
main_list.append(list2)
for list in main_list:
for string in list:
for word in string.split():
if word not in lookup_dict:
lookup_dict[word] = i
i += 1
return lookup_dict
def encode(input_list, input_dict):
encoded_list = []
for string in input_list:
sentence_list = []
for word in string.split():
sentence_list.append(input_dict[word]) # value lookup from dictionary.. int
encoded_list.append(sentence_list)
return encoded_list
def pad_data(list_of_lists):
padded_data = tf.keras.preprocessing.sequence.pad_sequences(list_of_lists, padding='post')
return padded_data
def create_array_sentiment_integers(list):
sent_int_list = []
for sentiment in list:
sent_int_list.append(sentiment)
return np.asarray(sent_int_list, dtype=np.int32)
X_train_stemmed_list = stemming_column(X_train_no_punc_lowercase['text'])
X_test_stemmed_list = stemming_column(X_test_no_punc_lowercase['text'])
lookup_table = create_lookup_table(X_train_stemmed_list, X_test_stemmed_list)
X_train_encoded_list = encode(X_train_stemmed_list, lookup_table)
X_train_padded_data = pad_data(X_train_encoded_list)
Y_train = create_array_sentiment_integers(train_no_punc_lowercase['sentiment'])
max_features = 3 # 3 choices 0, 1, 2
Y_train_final = np.zeros((Y_train.shape[0], max_features), dtype=np.float32)
Y_train_final[np.arange(Y_train.shape[0]), Y_train] = 1.0
input_dimension = len(lookup_table) + 1
output_dimension = 64
input_length = 33
model = Sequential()
model.add(tf.keras.layers.Embedding(input_dim=input_dimension,
output_dim=output_dimension,
input_length=input_length,
mask_zero=True))
model.add(tf.keras.layers.LSTM(512, dropout=0.2, recurrent_dropout=0.2, return_sequences=True))
model.add(tf.keras.layers.Dense(256, activation='sigmoid'))
model.add(tf.keras.layers.Dropout(0.2))
model.add(Dense(3, activation='softmax'))
model.compile(loss='binary_crossentropy',
optimizer='adam',
metrics=['accuracy'])
model.fit(X_train_padded_data, Y_train_final, validation_split=0.20, epochs=10)
model.save('Tweet_sentiment.model')
此外,這里是數據集的形狀..
x train shape: (27481, 33, 1) x train type: <class 'numpy.ndarray'> y train shape: (27481, 3)
錯誤代碼
Epoch 1/3
363/859 [===========>..................] - ETA: 9s - loss: 0.5449 - accuracy: 0.5674
---------------------------------------------------------------------------
UnknownError Traceback (most recent call last)
<ipython-input-103-1d4af3962607> in <module>()
----> 1 model.fit(X_train_padded_data, Y_train_final, epochs=3,)
8 frames
/usr/local/lib/python3.6/dist-packages/tensorflow/python/eager/execute.py in quick_execute(op_name, num_outputs, inputs, attrs, ctx, name)
58 ctx.ensure_initialized()
59 tensors = pywrap_tfe.TFE_Py_Execute(ctx._handle, device_name, op_name,
---> 60 inputs, attrs, num_outputs)
61 except core._NotOkStatusException as e:
62 if name is not None:
UnknownError: [_Derived_] CUDNN_STATUS_BAD_PARAM
in tensorflow/stream_executor/cuda/cuda_dnn.cc(1496): 'cudnnSetRNNDataDescriptor( data_desc.get(), data_type, layout, max_seq_length, batch_size, data_size, seq_lengths_array, (void*)&padding_fill)'
[[{{node cond_38/then/_0/CudnnRNNV3}}]]
[[sequential_5/lstm_4/StatefulPartitionedCall]] [Op:__inference_train_function_36098]
Function call stack:
train_function -> train_function -> train_function
我在您的代碼中看到了一些問題。 它們在下面提到:
您正在使用input_dimension = len(lookup_table) + 1
。 len(lookup_table)
只不過是Number of Time Steps
。 它的價值會很高,至少3萬多。 建議僅使用這些值的子集。 因此,您可以設置input_dimension = 10000
或input_dimension = 15000
(您可以嘗試使用此值)它應該可以解決問題。 話雖如此,它不會影響 Model 的精度。
為什么將Recurrent Dropout
設置為浮點值工作 ==> 當我們設置Recurrent Dropout
時,它實際上會降低Number of Time Steps
,在您的情況下為input_dimension
,因此它不會崩潰。
LSTM Layer
LSTM Layer
,才應使用return_sequences=True
。 由於您只有一個LSTM Layer
,因此return_sequences
應設置為False
binary_crossentropy
。 如果您不是One-Hot-Encoding
您的Target
或categorical_crossentropy
如果您是One-Hot-Encoding
您的Target
,則應該使用sparse_categorical_crossentropy
。Embedding Layer
中使用Masking
嗎? 此外,我看到您正在使用許多函數和許多代碼行進行Data-Preprocessing
,例如刪除Hyperlinks
、刪除Punctuations
、 Tokenizing
化等。
所以,我想我會提供一個End-To-End Tutorial
Text Classification
教程,它會幫助你以及Stack Overflow Community
。 相同的代碼如下所示:
#!pip install tensorflow==2.1
#!pip install nltk
#!pip install tika
#!pip install textblob
#!pip3 install --upgrade numpy
#!pip install scikit-learn
# To handle Paths
import os
# To remove Hyperlinks and Dates
import re
# To remove Puncutations
import string
# This helps to remove the unnecessary words from our Text Data
from nltk.corpus import stopwords
STOPWORDS = set(stopwords.words('english'))
# To Parse the Input Data Files
from tika import parser
from textblob import TextBlob
# In order to use the Libraries of Tensorflow
import tensorflow as tf
# For Preprocessing the Text => To Tokenize the Text
from tensorflow.keras.preprocessing.text import Tokenizer
# If the Two Articles are of different length, pad_sequences will make the length equal
from tensorflow.keras.preprocessing.sequence import pad_sequences
# Package for performing Numerical Operations
import numpy as np
# MatplotLib for Plotting Graphs
import matplotlib.pyplot as plt
# To shuffle the Data
from random import shuffle
# To Partition the Data into Train Data and Test Data
from sklearn.model_selection import train_test_split
# To add Regularizer in order to reduce Overfitting
from tensorflow.keras.regularizers import l2
# Give the Path of our Data
Path_Of_Data = 'Data'
# Extract the Labels from the Folders inside the Path mentioned above
Unique_Labels_List = ['negative', 'neutral', 'positive']
def GetNumericLabel(EachLabel):
if EachLabel=='negative':
return 0
elif EachLabel=='neutral':
return 1
elif EachLabel=='positive':
return 2
def Pre_Process_Data_And_Create_BOW(folder_path):
#creating empty lists in order to Create Resume Text and the respective Label
Resumes_List = []
Labels_List = []
for EachLabel in Unique_Labels_List:
for root, dirs, files in os.walk(os.path.join(folder_path, EachLabel),topdown=False):
for file in files:
i = 0
if file.endswith('.pdf'):
#Access individual file
Full_Resume_Path = os.path.join(root, file)
# Parse the Data inside the file
file_data = parser.from_file(Full_Resume_Path)
# Extract the Content of the File
Resume_Text = file_data['content']
# Below Code removes the Hyperlinks in the Resume, like LinkedIn Profile, Certifications, etc..
HyperLink_Regex = r'http[s]?://(?:[a-zA-Z]|[0-9]|[$-_@.&+]|[!*\(\), ]|(?:%[0-9a-fA-F][0-9a-fA-F]))+'
Text_Without_HL = re.sub(HyperLink_Regex, ' ', Resume_Text, flags=re.MULTILINE)
# Below Code removes the Date from the Resume
Date_regEx = r'(?:\d{1,2}[-/th|st|nd|rd\s]*)?(?:Jan|Feb|Mar|Apr|May|Jun|Jul|Aug|Sep|Oct|Nov|Dec)?[a-z\s,.]*(?:\d{1,2}[-/th|st|nd|rd)\s,]*)+(?:\d{2,4})+'
CleanedText = re.sub(Date_regEx,' ',Text_Without_HL)
List_Of_All_Punctuations = list(string.punctuation)
Important_Punctuations = ['#', '.', '+' , '-'] #Add more, if any other Punctuation is observed as Important
NewLineChar = '\n'
# Below Set Comprises all the Punctuations, which can be Removed from the Text of Resume
Total_Punct = len(List_Of_All_Punctuations)
for EachImpPunct in Important_Punctuations:
for CountOfPunct in range(Total_Punct):
if CountOfPunct == Total_Punct:
break
elif EachImpPunct == List_Of_All_Punctuations[CountOfPunct]:
del List_Of_All_Punctuations[CountOfPunct]
Total_Punct = Total_Punct - 1
List_Of_All_Punctuations.append(NewLineChar)
for EachPunct in List_Of_All_Punctuations:
CleanedText = CleanedText.replace(EachPunct, " ")
# Below Code converts all the Words in the Resume to Lowercase ======> Check if it has to come after Tokenization if Splitting Code is delet instead of integed
#Final_Cleaned_Resume_Text = Text_Without_Punct.lower()
Final_Cleaned_Resume_Text = CleanedText.lower()
#Code to remove Stopwords from each Resume
for word in STOPWORDS:
#stop_token = ' ' + word + ' '
stop_token = word
Resume_Text = Final_Cleaned_Resume_Text.replace(stop_token, ' ')
#Resume_Text = Resume_Text.replace(' ', ' ')
Resumes_List.append(Resume_Text)
Numeric_Label = GetNumericLabel(EachLabel)
Labels_List.append(Numeric_Label)
#print('Successfully executed for the Folder, ', EachLabel)
#Return Final Lists
return Resumes_List, Labels_List
#calling the function and passing the path
Resumes_List, Labels_List = Pre_Process_Data_And_Create_BOW(Path_Of_Data)
vocab_size = 10000 # This is very important for you
# We want the Output of the Embedding Layer to be 64
embedding_dim = 64
max_length = 800
trunc_type = 'post'
padding_type = 'post'
oov_tok = '<OOV>'
# Taking 80% of the Data as Training Data and remaining 20% will be for Test Data
training_portion = .8
# Size of Train Data is 80% of the Entire Dataset => 0.8 * 2225
Train_Resume_Size = int(len(Resumes_List) * training_portion)
Labels_List = np.asarray(Labels_List)
Train_Resume_Data, Validation_Resume_Data, Train_Labels, Validation_Labels = \
train_test_split(Resumes_List, Labels_List, train_size = training_portion,
shuffle = True
, stratify= Labels_List)
from statistics import mean
print('Average Number of Words in Each Training Resume is {}'.format(mean([len(i) for i in Train_Resume_Data])))
tokenizer = Tokenizer(num_words = vocab_size, oov_token=oov_tok)
tokenizer.fit_on_texts(Train_Resume_Data)
word_index = tokenizer.word_index
# Convert the Word Tokens into Integer equivalents, before passing it to keras embedding layer
train_sequences = tokenizer.texts_to_sequences(Train_Resume_Data)
train_padded = pad_sequences(train_sequences, maxlen=max_length, padding=padding_type, truncating=trunc_type)
validation_sequences = tokenizer.texts_to_sequences(Validation_Resume_Data)
validation_padded = pad_sequences(validation_sequences, maxlen=max_length, padding=padding_type, truncating=trunc_type)
print(len(validation_sequences))
print(validation_padded.shape)
reverse_word_index = dict([(value, key) for (key, value) in word_index.items()])
# Check your Data
def decode_article(text):
return ' '.join([reverse_word_index.get(i, '?') for i in text])
print(decode_article(train_padded[10]))
print('-------------------------------------------------------------------------')
print(Train_Resume_Data[10])
Regularizer = l2(0.001)
model = tf.keras.Sequential([
# Add an Embedding layer expecting input vocab of size 5000, and output embedding dimension of size 64 we set at the top
tf.keras.layers.Embedding(vocab_size, embedding_dim,
embeddings_regularizer = Regularizer),
tf.keras.layers.Bidirectional(tf.keras.layers.LSTM(embedding_dim, return_sequences=True)),
tf.keras.layers.Bidirectional(tf.keras.layers.LSTM(32)),
# use ReLU in place of tanh function since they are very good alternatives of each other.
tf.keras.layers.Dense(embedding_dim, activation='relu'),
# Add a Dense layer with 3 units and softmax activation.
# When we have multiple outputs, softmax convert outputs layers into a probability distribution.
tf.keras.layers.Dense(3, activation='softmax')
])
model.summary()
#Using Early Stopping in order to handle Overfitting
ES_Callback = tf.keras.callbacks.EarlyStopping(monitor='val_loss', patience=10)
model.compile(loss = tf.keras.losses.SparseCategoricalCrossentropy(), optimizer='adam', metrics=['accuracy'])
num_epochs = 100
history = model.fit(x = train_padded, y = Train_Labels, epochs=num_epochs,
callbacks=[ES_Callback],
validation_data=(validation_padded, Validation_Labels),
batch_size = 32, shuffle=True, verbose=1)
def plot_graphs(history, string):
plt.plot(history.history[string])
plt.plot(history.history['val_'+string])
plt.xlabel("Epochs")
plt.ylabel(string)
plt.legend([string, 'val_'+string])
plt.show()
plot_graphs(history, "accuracy")
plot_graphs(history, "loss")
version = 1
MODEL_DIR = 'Resume_Classification_Model'
export_path = os.path.join(MODEL_DIR, str(version))
tf.keras.models.save_model(model = model, filepath = export_path)
!ls -l {export_path}
!saved_model_cli show --dir {export_path} --all
欲了解更多信息,請參閱這篇美麗的文章。
希望這可以解決您的問題。 快樂學習!
聲明:本站的技術帖子網頁,遵循CC BY-SA 4.0協議,如果您需要轉載,請注明本站網址或者原文地址。任何問題請咨詢:yoyou2525@163.com.