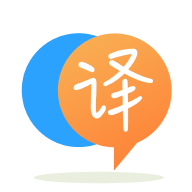
[英]InvalidArgumentError: logits and labels must have the same first dimension, got logits shape [1,10] and labels shape [40]
[英]InvalidArgumentError: logits and labels must have the same first dimension, got logits shape [15488,3] and labels shape [32]
我的代碼不工作我得到 InvalidArgumentError: logits and labels must have the same first dimension, got logits shape [15488,3] and labels shape [32] [[node sparse_categorical_crossentropy/SparseSoftmaxCrossEntropyWithLogits/SparseSoftmaxCrossEntropyWithLogits(定義在/usr/local/ lib/python3.7/dist-packages/keras/backend.py:5114) ]] [Op:__inference_train_function_2889]
AUTOTUNE = tf.data.AUTOTUNE
train_ds = train_ds.cache().shuffle(1000).prefetch(buffer_size=AUTOTUNE)
val_ds = val_ds.cache().prefetch(buffer_size=AUTOTUNE)
data_augmentation = tf.keras.Sequential([
tf.keras.layers.experimental.preprocessing.RandomFlip('horizontal'),
tf.keras.layers.experimental.preprocessing.RandomRotation(0.2),
])
for image, _ in train_ds.take(1):
plt.figure(figsize=(10, 10))
first_image = image[0]
for i in range(9):
ax = plt.subplot(3, 3, i + 1)
augmented_image = data_augmentation(tf.expand_dims(first_image, 0))
plt.imshow(augmented_image[0] / 255)
plt.axis('off')
normalization_layer = layers.experimental.preprocessing.Rescaling(1./255)
normalized_ds = train_ds.map(lambda x, y: (normalization_layer(x), y))
image_batch, labels_batch = next(iter(normalized_ds))
first_image = image_batch[0]
# Notice the pixels values are now in `[0,1]`.
print(np.min(first_image), np.max(first_image))
num_classes = 3
model = Sequential([
layers.experimental.preprocessing.Rescaling(1./255, input_shape=(img_height, img_width, 3)),
layers.MaxPooling2D(),
layers.Conv2D(32, 3, padding='same', activation='relu'),
layers.MaxPooling2D(),
layers.Conv2D(64, 3, padding='same', activation='relu'),
layers.MaxPooling2D(),
layers.Dense(128, activation='relu'),
layers.Dense(num_classes)
])
model.compile(optimizer='adam',
loss=tf.keras.losses.SparseCategoricalCrossentropy(from_logits=True),
metrics=['accuracy'])
model.summary()
epochs= 10
history = model.fit(
train_ds,
validation_data=val_ds,
epochs=epochs
)
acc = history.history['accuracy']
val_acc = history.history['val_accuracy']
loss = history.history['loss']
val_loss = history.history['val_loss']
epochs_range = range(epochs)
plt.figure(figsize=(8, 8))
plt.subplot(1, 2, 1)
plt.plot(epochs_range, acc, label='Training Accuracy')
plt.plot(epochs_range, val_acc, label='Validation Accuracy')
plt.legend(loc='lower right')
plt.title('Training and Validation Accuracy')
plt.subplot(1, 2, 2)
plt.plot(epochs_range, loss, label='Training Loss')
plt.plot(epochs_range, val_loss, label='Validation Loss')
plt.legend(loc='upper right')
plt.title('Training and Validation Loss')
plt.show()
在你的代碼之后
layers.MaxPooling2D()
您需要在將數據應用到密集層之前將其展平,因此添加
layers.Flatten()
聲明:本站的技術帖子網頁,遵循CC BY-SA 4.0協議,如果您需要轉載,請注明本站網址或者原文地址。任何問題請咨詢:yoyou2525@163.com.