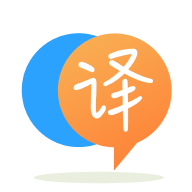
[英]how can fixed parameters cost and gamma using libsvm matlab to improve accuracy?
[英]Matlab parameters selction using Balance Accuracy of Libsvm
我正在尝试合并这两位代码。 我试图使用平衡精度(BAC)而不是精度来进行参数选择。 我已经在libsvm上下载了补充文件以处理平衡精度。
我用于参数选择的代码是:
prompt ='CROSS VALIDATION MAXIMUM STEP RANGE) ? ';
maxstep = input (prompt);
stepSize = 1;
log2c_list = -maxstep:stepSize:maxstep;
log2g_list = -maxstep:stepSize:maxstep;
maxc = max(log2c_list);
maxg = max(log2c_list);
numLog2c = length(log2c_list);
numLog2g = length(log2g_list);
cvMatrix = zeros(numLog2c,numLog2g);
bestcv = 0;
for i = 1:numLog2c
log2c = log2c_list(i);
for j = 1:numLog2g
log2g = log2g_list(j);
% -v 3 --> 3-fold cross validation
param = ['-q -v 3 -c ', num2str(2^log2c), ' -g ', num2str(2^log2g)];
cv = svmtrain(class_vector_train, predictors_matrix_train, param);
cvMatrix(i,j) = cv;
if (cv >= bestcv),
bestcv = cv; bestLog2c = log2c; bestLog2g = log2g;
end
% fprintf('%g %g %g (best c=%g, g=%g, rate=%g)\n', log2c, log2g, cv, bestc, bestg, bestcv);
end
end
disp(['CV scale1: best log2c:',num2str(bestLog2c),' best log2g:',num2str(bestLog2g),' accuracy:',num2str(bestcv),'%']);
为了获得我使用的天平精度:
do_binary_cross_validation(class_vector_train, predictors_matrix_train,'-c 1 -g 2',5);
model = svmtrain(class_vector_train, predictors_matrix_train);
[predicted_class_test, evaluation_results, decision_values] = do_binary_predict(class_vector_test, predictors_matrix_test, model);
但是我找不到平衡的精度度量。
使用功能bac
您的validate_function.m文件:
function ret = bac(dec, label)
tp = sum(label == 1 & dec >= 0);
tn = sum(label == -1 & dec < 0);
tp_fn = sum(label == 1);
tn_fp = sum(label == -1);
if tp_fn == 0;
disp(sprintf('warning: No positive true label.'));
sensitivity = 0;
else
sensitivity = tp / tp_fn;
end
if tn_fp == 0;
disp(sprintf('warning: No negative true label.'));
specificity = 0;
else
specificity = tn / tn_fp;
end
ret = (sensitivity + specificity) / 2;
disp(sprintf('BAC = %g', ret));
这将计算BAC (Balanced ACcuracy) = (Sensitivity + Specificity) / 2
,其中Sensitivity = true_positive / (true_positive + false_negative)
和Specificity = true_negative / (true_negative + false_positive)
声明:本站的技术帖子网页,遵循CC BY-SA 4.0协议,如果您需要转载,请注明本站网址或者原文地址。任何问题请咨询:yoyou2525@163.com.