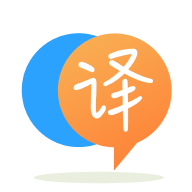
[英]How to get a single value from softmax instead of probability & get confusion matrix
[英]How to vectorize Softmax probability of a multi dimensional matrix
我正在尝试完成斯坦福cs244n类的作业1 。 问题1b强烈建议对Softmax函数进行优化。 我设法获得了N维向量的Softmax。 我还获得了MxN维矩阵的Softmax,但使用了for循环遍历各列。 我有以下代码:
def softmax(x):
orig_shape = x.shape
# Matrix
if len(x.shape) > 1:
softmax = np.zeros(orig_shape)
for i,col in enumerate(x):
softmax[i] = np.exp(col - np.max(col))/np.sum(np.exp(col - np.max(col)))
# Vector
else:
softmax = np.exp(x - np.max(x))/np.sum(np.exp(x - np.max(x)))
return softmax
我可以实施更优化的Matrix实施吗?
在相关的ufuncs
上使用NumPy broadcasting
,该NumPy broadcasting
涵盖了具有通用维数的ndarray-
exp_max = np.exp(x - np.max(x,axis=-1,keepdims=True))
out = exp_max/np.sum(exp_max,axis=-1,keepdims=True)
您可以尝试使用np.apply_along_axis
,在其中必须指定执行代码的axis=1
(在您的情况下, axis=1
)。 这是一个工作示例:
In [1]: import numpy as np
In [2]: def softmax(x):
...: orig_shape = x.shape
...:
...: # Matrix
...: if len(x.shape) > 1:
...: softmax = np.zeros(orig_shape)
...: for i,col in enumerate(x):
...: softmax[i] = np.exp(col - np.max(col))/np.sum(np.exp(col - np.max(col)))
...: # Vector
...: else:
...: softmax = np.exp(x - np.max(x))/np.sum(np.exp(x - np.max(x)))
...: return softmax
...:
In [3]: def softmax_vectorize(x):
...: return np.exp(x - np.max(x))/np.sum(np.exp(x - np.max(x)))
...:
In [4]: X = np.array([[1, 0, 0, 4, 5, 0, 7],
...: [1, 0, 0, 4, 5, 0, 7],
...: [1, 0, 0, 4, 5, 0, 7]])
In [5]: print softmax(X)
[[ 2.08239574e-03 7.66070581e-04 7.66070581e-04 4.18260365e-02
1.13694955e-01 7.66070581e-04 8.40098401e-01]
[ 2.08239574e-03 7.66070581e-04 7.66070581e-04 4.18260365e-02
1.13694955e-01 7.66070581e-04 8.40098401e-01]
[ 2.08239574e-03 7.66070581e-04 7.66070581e-04 4.18260365e-02
1.13694955e-01 7.66070581e-04 8.40098401e-01]]
In [6]: print np.apply_along_axis(softmax_vecorize, axis=1, arr=X)
[[ 2.08239574e-03 7.66070581e-04 7.66070581e-04 4.18260365e-02
1.13694955e-01 7.66070581e-04 8.40098401e-01]
[ 2.08239574e-03 7.66070581e-04 7.66070581e-04 4.18260365e-02
1.13694955e-01 7.66070581e-04 8.40098401e-01]
[ 2.08239574e-03 7.66070581e-04 7.66070581e-04 4.18260365e-02
1.13694955e-01 7.66070581e-04 8.40098401e-01]]
声明:本站的技术帖子网页,遵循CC BY-SA 4.0协议,如果您需要转载,请注明本站网址或者原文地址。任何问题请咨询:yoyou2525@163.com.