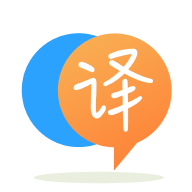
[英]compute the recall precision and F1 score for a multi label classification keras
[英]Getting precision, recall and F1 score per class in Keras
我在Keras(2.1.5)中使用TensorFlow后端训练了一个神经网络,我还使用了keras-contrib(2.0.8)库来添加CRF层作为网络的输出。
我想知道在使用NN对测试集进行预测后,如何获得每个类的精度,召回率和f1分数。
假设你有一个函数get_model()
,它构建了你训练过的完全相同的模型,以及指向包含模型权重的HDF5文件的路径weights_path
:
model = get_model()
model.load_weights(weights_path)
这应该正确加载您的模型。 然后,您只需定义测试数据的ImageDataGenerator
并拟合模型以获取预测:
# Path to your folder testing data
testing_folder = ""
# Image size (set up the image size used for training)
img_size = 256
# Batch size (you should tune it based on your memory)
batch_size = 16
val_datagen = ImageDataGenerator(
rescale=1. / 255)
validation_generator = val_datagen.flow_from_directory(
testing_folder,
target_size=(img_size, img_size),
batch_size=batch_size,
shuffle=False,
class_mode='categorical')
然后,您可以使用model.predict_generator()
方法使模型生成整个数据集的所有预测:
# Number of steps corresponding to an epoch
steps = 100
predictions = model.predict_generator(validation_generator, steps=steps)
最后使用sklearn
包中的metrics.confusion_matrix()
方法创建一个confussion矩阵:
val_preds = np.argmax(predictions, axis=-1)
val_trues = validation_generator.classes
cm = metrics.confusion_matrix(val_trues, val_preds)
或者让所有精度,回顾和F1-分数使用的所有类metrics.precision_recall_fscore_support()
方法从sklearn
(自变量average=None
输出度量的所有类):
# label names
labels = validation_generator.class_indices.keys()
precisions, recall, f1_score, _ = metrics.precision_recall_fscore_support(val_trues, val_preds, labels=labels)
我没有测试过,但我猜这会对你有所帮助。
看看sklearn.metrics.classification_report
:
from sklearn.metrics import classification_report
y_pred = model.predict(x_test)
print(classification_report(y_true, y_pred))
给你一些类似的东西
precision recall f1-score support
class 0 0.50 1.00 0.67 1
class 1 0.00 0.00 0.00 1
class 2 1.00 0.67 0.80 3
avg / total 0.70 0.60 0.61 5
声明:本站的技术帖子网页,遵循CC BY-SA 4.0协议,如果您需要转载,请注明本站网址或者原文地址。任何问题请咨询:yoyou2525@163.com.