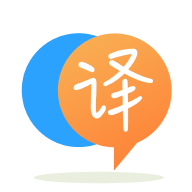
[英]Implement Custom Cost function in keras which requires output of whole batch-size
[英]How to implement a custom cost function in keras?
我有以下成本函数 = argmin L1+L2,其中 L1 是均方误差,L2 是 -λ Summation( Square((y) x (z) )) 其中 y 是预测的 output 图像,z 是给定的输入图像至 model。 y 和 z 的元素乘法,然后取平方。 λ 是 L1 和 L2 之间的权衡参数。 我不知道如何实现,我做了如下
def custom_loss(i):
def loss(y_true, y_pred):
y_true=K.cast(y_true, dtype='float32')
y_pred=K.cast(y_pred, dtype='float32')
input_image=K.cast(i, dtype='float32')
mul=tf.math.multiply(input_image,y_pred)
L1=K.mean(K.square(mul),axis=1)
L2=K.mean(K.square(y_pred - y_true), axis=-1)
closs=L1-L2
return closs
return loss
一部分一部分地打破你的问题
其中 L1 是均方误差
因此, L1 = np.square(np.subtract(y_true,y_pred)).mean()
L2 是 -λ Summation( Square((y) x (z) )),其中 y 是预测的 output 图像,z 是 model 的给定输入图像。 y和z的元素乘法,然后取平方
因此, L2 = np.sum(np.concatenate(np.square(np.multiply(y_true,y_pred))))
。 您意识到 L2 将是一个非常大的损失数字。
总结一下,这就是您的损失 function 的样子 -
def custom_loss(y_true,y_pred):
def loss(y_true, y_pred):
y_true = img_to_array(y_true)
y_pred = img_to_array(y_pred)
L1 = np.square(np.subtract(y_true,y_pred)).mean()
L2 = np.sum(np.concatenate(np.square(np.multiply(y_true,y_pred))))
loss=L1-L2
return loss
我在这里编写了一个简单的代码来将图像加载为 y_true 并裁剪 y_pred 的中心部分并执行您提到的损失(没有太大意义,因为值很大) 。
代码 -
import tensorflow as tf
from keras.preprocessing.image import load_img
from keras.preprocessing.image import img_to_array, array_to_img
from matplotlib import pyplot
# Load the image
y_true = load_img('/content/bird.jpg', target_size=(224, 224))
# Convert Image to array
image = img_to_array(y_true)
# Central crop image
image = tf.image.central_crop(image, np.random.uniform(0.50, 1.00))
# Resize to original size of image
image = tf.image.resize(image, (224, 224))
# convert the image to an array
y_pred = array_to_img(image)
# def custom_loss():
# def loss(y_true, y_pred):
# y_true = img_to_array(y_true)
# y_pred = img_to_array(y_pred)
# L1 = np.square(np.subtract(y_true,y_pred)).mean()
# L2 = np.sum(np.concatenate(np.square(np.multiply(y_true,y_pred))))
# loss=L1-L2
# return loss
def loss(y_true, y_pred):
y_true = img_to_array(y_true)
y_pred = img_to_array(y_pred)
L1 = np.square(np.subtract(y_true,y_pred)).mean()
L2 = np.sum(np.concatenate(np.square(np.multiply(y_true,y_pred))))
loss=L1-L2
return loss
x = loss(y_true,y_pred)
print(x)
Output -
-251577020000000.0
声明:本站的技术帖子网页,遵循CC BY-SA 4.0协议,如果您需要转载,请注明本站网址或者原文地址。任何问题请咨询:yoyou2525@163.com.