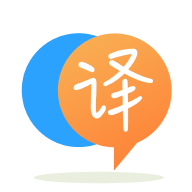
[英]Image classification with tensorflow: Three different methods evaluate the result with same model and same test data but yield different results
[英]Classification of the same image gives different result
我正在尝试使用带有 Tensorflow 的 CNN 对 3D 图像进行分类,我认为有问题。 训练和验证后,我使用保存的 model 对单个图像进行分类。 当我对这张图片进行分类时,每次尝试都会给我不同的结果。
例子:
1st try:
input: Picture of a 3D brain
result of the classification: 1
2nd try:
input: Same picture of 3D brain
result of the classification: 2
我想知道这是正常的还是我做错了什么。 我正在使用的 model 的准确度为 67%。
这是代码:
def conv3d(x, W):
return tf.nn.conv3d(x, W, strides=[1, 1, 1, 1, 1], padding='SAME')
def maxpool3d(x):
return tf.nn.max_pool3d(x, ksize=[1, 2, 2, 2, 1], strides=[1, 2, 2, 2, 1], padding='SAME')
def convolutional_neural_network(x):
number = calc()
weights = {'W_conv1': tf.Variable(tf.random_normal([3, 3, 3, 1, 32])),
'W_conv2': tf.Variable(tf.random_normal([3, 3, 3, 32, 64])),
'W_fc': tf.Variable(tf.random_normal([number, 1024])),
'out': tf.Variable(tf.random_normal([1024, 3]))}
biases = {'b_conv1': tf.Variable(tf.random_normal([32])),
'b_conv2': tf.Variable(tf.random_normal([64])),
'b_fc': tf.Variable(tf.random_normal([1024])),
'out': tf.Variable(tf.random_normal([3]))}
x = tf.reshape(x, shape=[-1, 50, 50, 30, 1])
conv1 = tf.nn.relu(conv3d(x, weights['W_conv1']) + biases['b_conv1'])
conv1 = maxpool3d(conv1)
conv2 = tf.nn.relu(conv3d(conv1, weights['W_conv2']) + biases['b_conv2'])
conv2 = maxpool3d(conv2)
fc = tf.reshape(conv2, [-1, number])
fc = tf.nn.relu(tf.matmul(fc, weights['W_fc']) + biases['b_fc'])
fc = tf.nn.dropout(fc, 0.8)
output = tf.matmul(fc, weights['out']) + biases['out']
return output
那就是主要的function:
def classification(path):
x = tf.placeholder('float')
new_path = extract(path + '.gz')
X_new = process_data(path=new_path, apply_hist=True)
pred = convolutional_neural_network(x)
res = 0
with tf.Session() as sess:
saver = tf.train.import_meta_graph('modelo.meta')
saver.restore(sess, 'modelo')
sess.run(tf.initialize_all_variables())
probabilities = tf.nn.softmax(pred)
c = sess.run(probabilities, feed_dict={x: X_new})
res = np.argmax(c)
return res
我正在对输入图像进行相同的处理。
太感谢了!
编辑:
我尝试交换这些行,但结果仍然不同。
with tf.Session() as sess:
sess.run(tf.initialize_all_variables())
saver = tf.train.import_meta_graph('modelo.meta')
saver.restore(sess, 'modelo')
您首先恢复变量,然后将变量初始化为随机值:
saver.restore(sess, 'modelo')
sess.run(tf.initialize_all_variables())
因此,您有两个不同的预测,因为您使用随机变量两次初始化 model。 您需要交换这些行,或省略 initialize_all_vars 总括。 老实说,我不知道如何解决它,但至少这会让你走上正确的道路。 希望这可以帮助!
声明:本站的技术帖子网页,遵循CC BY-SA 4.0协议,如果您需要转载,请注明本站网址或者原文地址。任何问题请咨询:yoyou2525@163.com.