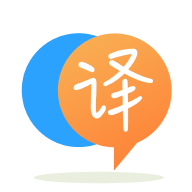
[英]Tensorflow 1.15 is not loading frozen graph, and has given me the same error for the past week
[英]Error while exporting interference graph in Tensorflow 1.15
我在尝试导出训练有素的模型(调整为 1500 步)时遇到以下错误:
Traceback (most recent call last):
File "export_inference_graph.py", line 150, in <module>
tf.app.run()
File "C:\Users\USERNAME\anaconda3\envs\test123\lib\site-packages\tensorflow_core\python\platform\app.py", line 40, in run
_run(main=main, argv=argv, flags_parser=_parse_flags_tolerate_undef)
File "C:\Users\USERNAME\anaconda3\envs\test123\lib\site-packages\absl\app.py", line 312, in run
_run_main(main, args)
File "C:\Users\USERNAME\anaconda3\envs\test123\lib\site-packages\absl\app.py", line 258, in _run_main
sys.exit(main(argv))
File "export_inference_graph.py", line 146, in main
write_inference_graph=FLAGS.write_inference_graph)
File "C:\Users\USERNAME\Desktop\DirectML_Tensorflow_Library\Tensorflow\workspace\training_demo\object_detection\exporter.py", line 455, in export_inference_graph
write_inference_graph=write_inference_graph)
File "C:\Users\USERNAME\Desktop\DirectML_Tensorflow_Library\Tensorflow\workspace\training_demo\object_detection\exporter.py", line 384, in _export_inference_graph
trained_checkpoint_prefix=checkpoint_to_use)
File "C:\Users\USERNAME\Desktop\DirectML_Tensorflow_Library\Tensorflow\workspace\training_demo\object_detection\exporter.py", line 295, in write_graph_and_checkpoint
saver.restore(sess, trained_checkpoint_prefix)
File "C:\Users\USERNAME\anaconda3\envs\test123\lib\site-packages\tensorflow_core\python\training\saver.py", line 1282, in restore
checkpoint_prefix)
ValueError: The passed save_path is not a valid checkpoint: C:\\Users\\USERNAME\\Desktop\\DirectML_Tensorflow_Library\\Tensorflow\\workspace\\training_demo\\my_models\\my_ssd_mobilenet_v1_coco_2018_01_28\\checkpoints\\model.ckpt-1500
这是我设置 model 时的配置路径:
model {
ssd {
num_classes: 1
image_resizer {
fixed_shape_resizer {
height: 300
width: 300
}
}
feature_extractor {
type: "ssd_mobilenet_v1"
depth_multiplier: 1.0
min_depth: 16
conv_hyperparams {
regularizer {
l2_regularizer {
weight: 3.99999989895e-05
}
}
initializer {
truncated_normal_initializer {
mean: 0.0
stddev: 0.0299999993294
}
}
activation: RELU_6
batch_norm {
decay: 0.999700009823
center: true
scale: true
epsilon: 0.0010000000475
train: true
}
}
override_base_feature_extractor_hyperparams: true
}
box_coder {
faster_rcnn_box_coder {
y_scale: 10.0
x_scale: 10.0
height_scale: 5.0
width_scale: 5.0
}
}
matcher {
argmax_matcher {
matched_threshold: 0.5
unmatched_threshold: 0.5
ignore_thresholds: false
negatives_lower_than_unmatched: true
force_match_for_each_row: true
}
}
similarity_calculator {
iou_similarity {
}
}
box_predictor {
convolutional_box_predictor {
conv_hyperparams {
regularizer {
l2_regularizer {
weight: 3.99999989895e-05
}
}
initializer {
truncated_normal_initializer {
mean: 0.0
stddev: 0.0299999993294
}
}
activation: RELU_6
batch_norm {
decay: 0.999700009823
center: true
scale: true
epsilon: 0.0010000000475
train: true
}
}
min_depth: 0
max_depth: 0
num_layers_before_predictor: 0
use_dropout: false
dropout_keep_probability: 0.800000011921
kernel_size: 1
box_code_size: 4
apply_sigmoid_to_scores: false
}
}
anchor_generator {
ssd_anchor_generator {
num_layers: 6
min_scale: 0.20000000298
max_scale: 0.949999988079
aspect_ratios: 1.0
aspect_ratios: 2.0
aspect_ratios: 0.5
aspect_ratios: 3.0
aspect_ratios: 0.333299994469
}
}
post_processing {
batch_non_max_suppression {
score_threshold: 0.300000011921
iou_threshold: 0.600000023842
max_detections_per_class: 100
max_total_detections: 100
}
score_converter: SIGMOID
}
normalize_loss_by_num_matches: true
loss {
localization_loss {
weighted_smooth_l1 {
}
}
classification_loss {
weighted_sigmoid {
}
}
hard_example_miner {
num_hard_examples: 3000
iou_threshold: 0.990000009537
loss_type: CLASSIFICATION
max_negatives_per_positive: 3
min_negatives_per_image: 0
}
classification_weight: 1.0
localization_weight: 1.0
}
}
}
train_config {
batch_size: 12
data_augmentation_options {
random_horizontal_flip {
}
}
data_augmentation_options {
ssd_random_crop {
}
}
optimizer {
rms_prop_optimizer {
learning_rate {
exponential_decay_learning_rate {
initial_learning_rate: 0.00400000018999
decay_steps: 800720
decay_factor: 0.949999988079
}
}
momentum_optimizer_value: 0.899999976158
decay: 0.899999976158
epsilon: 1.0
}
}
fine_tune_checkpoint: "C:\\Users\\USERNAME\\Desktop\\DirectML_Tensorflow_Library\\Tensorflow\\workspace\\training_demo\\pre-trained-model\\ssd_mobilenet_v1_coco_2018_01_28\\model.ckpt"
from_detection_checkpoint: true
num_steps: 1500
}
train_input_reader {
label_map_path: "C:\\Users\\USERNAME\\Desktop\\DirectML_Tensorflow_Library\\Tensorflow\\workspace\\training_demo\\annotations\\label_map.pbtxt"
tf_record_input_reader {
input_path: "C:\\Users\\USERNAME\\Desktop\\DirectML_Tensorflow_Library\\Tensorflow\\workspace\\training_demo\\annotations\\train.record"
}
}
eval_config {
num_examples: 540
max_evals: 10
use_moving_averages: false
}
eval_input_reader {
label_map_path: "C:\\Users\\USERNAME\\Desktop\\DirectML_Tensorflow_Library\\Tensorflow\\workspace\\training_demo\\annotations\\label_map.pbtxt"
shuffle: false
num_readers: 1
tf_record_input_reader {
input_path: "C:\\Users\\USERNAME\\Desktop\\DirectML_Tensorflow_Library\\Tensorflow\\workspace\\training_demo\\annotations\\test.record"
}
}
我已经用https://github.com/tensorflow/models/blob/master/research/object_detection/g3doc/tf1_detection_zoo.md#coco-trained-models中的一些其他模型训练了相同的数据集,并且再次发生相同的错误。
我的检查点文件夹包含“model.ckpt-1500.data-00000-of-00001”、“model.ckpt-1500.index”、“model.ckpt-1500.meta”和“检查点”。 在“检查点”中,model_checkpoint_path:“model.ckpt-1500”。
所以检查点存在,但是当我尝试导出它时,它不会被识别为有效的检查点。
我已经解决了我的问题,我在这里发布我的答案,以防将来可能会帮助其他人在设置 directml 1.15.5 和使用 model 检测动物园中的预训练模型时遇到同样的问题。
Go 进入 anaconda3 --> envs --> [您的 tensorflow 环境的名称; 在我的情况下 test123] --> Lib --> 站点包 --> tensorflow_estimator --> python --> 估计器 --> run_config.py
将 save_checkpoints_steps = None 更改为 save_checkpoints_steps = 100 (或您选择的任何数量),然后为了完成这项工作,您还需要将 save_checkpoints_secs = 600 更改为 save_checkpoints_secs = None
所以问题是文件“run_config.py”没有设置为保存我的检查点。
声明:本站的技术帖子网页,遵循CC BY-SA 4.0协议,如果您需要转载,请注明本站网址或者原文地址。任何问题请咨询:yoyou2525@163.com.