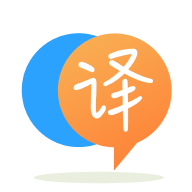
[英]String Matching Using TF-IDF, NGrams and Cosine Similarity in Python
[英]Matching Company names in Python through Cosine Similarity, TF-IDF and pyspark
我試圖將兩個列表中的公司名稱匹配在一起,以便檢查列表A中的公司是否確實在列表B中列出。由於公司名稱的書寫形式多種多樣,我傾向於使用cosing相似。 為此,我遵循了Ran Tavory在此博客上的注釋: Link Here
以下是一般概述:
- 在驅動程序上計算TF-IDF矩陣。
- 並行化矩陣A; 廣播矩陣B
- 現在,每個工作人員通過將其矩陣A的塊與整個矩陣B相乘來平整其工作塊。因此,如果一個工作人員在A [0:99]上進行操作,則它將這100行相乘並返回結果,例如A [13 ]匹配在B [21]中找到的名稱。 乘法是使用numpy完成的。
- 驅動程序將收集來自不同工作人員的所有結果,並將索引(A [13]和B [21])與原始數據集中的實際名稱進行匹配-我們完成了!
我能夠運行注釋中描述的確切代碼,但是其中一部分似乎有點奇怪: b_mat_dist = broadcast_matrix(a_mat)
在廣播a_mat以及並行化a_mat時,我得到了每個公司名稱都完全匹配的邏輯結果(因為我們正在尋找相同的來源)。
當我嘗試廣播b_mat時:b_mat_dist = broadcast_matrix(b_mat),出現以下錯誤: Incompatible dimension for X and Y matrices: X.shape[1] == 56710 while Y.shape[1] == 2418
任何幫助將不勝感激! 提前致謝 !
這是我的代碼:
import pandas as pd
from sklearn.feature_extraction.text import TfidfVectorizer
from sklearn.metrics.pairwise import cosine_similarity
from pyspark.sql import SQLContext, SparkSession
from pyspark import SparkContext
from scipy.sparse import csr_matrix
vectorizer = TfidfVectorizer()
if 'sc' in locals():
sc.stop()
sc = SparkContext("local", "Simple App")
pd.set_option('display.max_colwidth', -1)
RefB = pd.read_excel('Ref.xlsx')
ToMatchB = pd.read_excel('ToMatch.xlsx')
Ref = RefB['CLT_company_name']
ToMatch = ToMatchB ['Name1']
a_mat = vectorizer.fit_transform(Ref)
b_mat = vectorizer.fit_transform(ToMatch)
def find_matches_in_submatrix(sources, targets, inputs_start_index,
threshold=.8):
cosimilarities = cosine_similarity(sources, targets)
for i, cosimilarity in enumerate(cosimilarities):
cosimilarity = cosimilarity.flatten()
# Find the best match by using argsort()[-1]
target_index = cosimilarity.argsort()[-1]
source_index = inputs_start_index + i
similarity = cosimilarity[target_index]
if cosimilarity[target_index] >= threshold:
yield (source_index, target_index, similarity)
def broadcast_matrix(mat):
bcast = sc.broadcast((mat.data, mat.indices, mat.indptr))
(data, indices, indptr) = bcast.value
bcast_mat = csr_matrix((data, indices, indptr), shape=mat.shape)
return bcast_mat
def parallelize_matrix(scipy_mat, rows_per_chunk=100):
[rows, cols] = scipy_mat.shape
i = 0
submatrices = []
while i < rows:
current_chunk_size = min(rows_per_chunk, rows - i)
submat = scipy_mat[i:i + current_chunk_size]
submatrices.append((i, (submat.data, submat.indices,
submat.indptr),
(current_chunk_size, cols)))
i += current_chunk_size
return sc.parallelize(submatrices)
a_mat_para = parallelize_matrix(a_mat, rows_per_chunk=100)
b_mat_dist = broadcast_matrix(b_mat)
results = a_mat_para.flatMap(
lambda submatrix:
find_matches_in_submatrix(csr_matrix(submatrix[1],
shape=submatrix[2]),
b_mat_dist,
submatrix[0]))
嘗試均衡兩個TfidVectorizer對象的詞匯量:
vect = CountVectorizer()
vocabulary = vect.fit(Ref + ToMatch).vocabulary_
vectorizer = TfidfVectorizer(vocabulary=vocabulary)
同樣基於您的目標是:
a_mat = vectorizer.fit_transform(ToMatch)
b_mat = vectorizer.fit_transform(Ref)
對我來說似乎是一個更好的選擇。
聲明:本站的技術帖子網頁,遵循CC BY-SA 4.0協議,如果您需要轉載,請注明本站網址或者原文地址。任何問題請咨詢:yoyou2525@163.com.