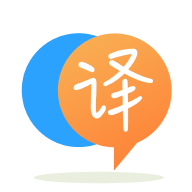
[英]Pytorch nn.CrossEntropyLoss giving, ValueError: Expected target size (x, y), got torch.Size([x, z]) for 3d tensor
[英]Pytorch ValueError: Expected target size (2, 13), got torch.Size([2]) when calling CrossEntropyLoss
我正在嘗試訓練 Pytorch LSTM 網絡,但是當我嘗試計算 CrossEntropyLoss 時出現ValueError: Expected target size (2, 13), got torch.Size([2])
。 我想我需要在某個地方改變形狀,但我不知道在哪里。
這是我的網絡定義:
class LSTM(nn.Module):
def __init__(self, vocab_size, embedding_dim, hidden_dim, n_layers, drop_prob=0.2):
super(LSTM, self).__init__()
# network size parameters
self.n_layers = n_layers
self.hidden_dim = hidden_dim
self.vocab_size = vocab_size
self.embedding_dim = embedding_dim
# the layers of the network
self.embedding = nn.Embedding(self.vocab_size, self.embedding_dim)
self.lstm = nn.LSTM(self.embedding_dim, self.hidden_dim, self.n_layers, dropout=drop_prob, batch_first=True)
self.dropout = nn.Dropout(drop_prob)
self.fc = nn.Linear(self.hidden_dim, self.vocab_size)
def forward(self, input, hidden):
# Perform a forward pass of the model on some input and hidden state.
batch_size = input.size(0)
print(f'batch_size: {batch_size}')
print(Input shape: {input.shape}')
# pass through embeddings layer
embeddings_out = self.embedding(input)
print(f'Shape after Embedding: {embeddings_out.shape}')
# pass through LSTM layers
lstm_out, hidden = self.lstm(embeddings_out, hidden)
print(f'Shape after LSTM: {lstm_out.shape}')
# pass through dropout layer
dropout_out = self.dropout(lstm_out)
print(f'Shape after Dropout: {dropout_out.shape}')
#pass through fully connected layer
fc_out = self.fc(dropout_out)
print(f'Shape after FC: {fc_out.shape}')
# return output and hidden state
return fc_out, hidden
def init_hidden(self, batch_size):
#Initializes hidden state
# Create two new tensors `with sizes n_layers x batch_size x hidden_dim,
# initialized to zero, for hidden state and cell state of LSTM
hidden = (torch.zeros(self.n_layers, batch_size, self.hidden_dim), torch.zeros(self.n_layers, batch_size, self.hidden_dim))
return hidden
我添加了注釋,說明了每個位置的網絡形狀。 我的數據位於名為 training_dataset 的 TensorDataset 中,具有兩個屬性、特征和標簽。 特征的形狀為 torch.Size([97, 3]),標簽的形狀為:torch.Size([97])。
這是網絡訓練的代碼:
# Size parameters
vocab_size = 13
embedding_dim = 256
hidden_dim = 256
n_layers = 2
# Training parameters
epochs = 3
learning_rate = 0.001
clip = 1
batch_size = 2
training_loader = DataLoader(training_dataset, batch_size=batch_size, drop_last=True, shuffle=True)
net = LSTM(vocab_size, embedding_dim, hidden_dim, n_layers)
optimizer = torch.optim.Adam(net.parameters(), lr=learning_rate)
loss_func = torch.nn.CrossEntropyLoss()
net.train()
for e in range(epochs):
print(f'Epoch {e}')
print(batch_size)
hidden = net.init_hidden(batch_size)
# loops through each batch
for features, labels in training_loader:
# resets training history
hidden = tuple([each.data for each in hidden])
net.zero_grad()
# computes gradient of loss from backprop
output, hidden = net.forward(features, hidden)
loss = loss_func(output, labels)
loss.backward()
# using clipping to avoid exploding gradient
nn.utils.clip_grad_norm_(net.parameters(), clip)
optimizer.step()
當我嘗試進行培訓時,出現以下錯誤:
Traceback (most recent call last):
File "train.py", line 75, in <module>
loss = loss_func(output, labels)
File "/usr/local/lib/python3.8/site-packages/torch/nn/modules/module.py", line 722, in _call_impl
result = self.forward(*input, **kwargs)
File "/usr/local/lib/python3.8/site-packages/torch/nn/modules/loss.py", line 947, in forward
return F.cross_entropy(input, target, weight=self.weight,
File "/usr/local/lib/python3.8/site-packages/torch/nn/functional.py", line 2422, in cross_entropy
return nll_loss(log_softmax(input, 1), target, weight, None, ignore_index, None, reduction)
File "/usr/local/lib/python3.8/site-packages/torch/nn/functional.py", line 2227, in nll_loss
raise ValueError('Expected target size {}, got {}'.format(
ValueError: Expected target size (2, 13), got torch.Size([2])
這里也是打印語句的結果:
batch_size: 2
Input shape: torch.Size([2, 3])
Shape after Embedding: torch.Size([2, 3, 256])
Shape after LSTM: torch.Size([2, 3, 256])
Shape after Dropout: torch.Size([2, 3, 256])
Shape after FC: torch.Size([2, 3, 13])
發生了某種形狀錯誤,但我不知道在哪里。 任何幫助,將不勝感激。 如果相關,我正在使用 Python 3.8.5 和 Pytorch 1.6.0。
對於將來遇到此問題的任何人,我在 pytorch 論壇上提出了同樣的問題,並得到了很好的答案,感謝 ptrblock,在此處找到。
問題是我的 LSTM 層有 batch_first=True,這意味着它返回輸入序列的每個成員的輸出(大小為 (batch_size, sequence_size, vocab_size))。 但是,我只想要輸入序列的最后一個成員的 output(大小為(batch_size,vocab_size)。
所以,在我的轉發 function 中,而不是
# pass through LSTM layers
lstm_out, hidden = self.lstm(embeddings_out, hidden)
它應該是
# pass through LSTM layers
lstm_out, hidden = self.lstm(embeddings_out, hidden)
# slice lstm_out to just get output of last element of the input sequence
lstm_out = lstm_out[:, -1]
這解決了形狀問題。 錯誤消息有點誤導,因為它說目標形狀錯誤,而實際上 output 形狀錯誤。
'預期目標大小 {},得到 {}',目標大小錯誤,請從 train_dataloader 檢查您的標簽。
聲明:本站的技術帖子網頁,遵循CC BY-SA 4.0協議,如果您需要轉載,請注明本站網址或者原文地址。任何問題請咨詢:yoyou2525@163.com.