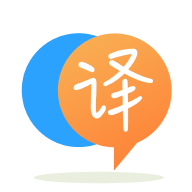
[英]PyTorch: Load/unpickle only the state dict of a model saved with torch.save
[英]torch.save(model.state_dict()) line comes error while model complated train and trying to save it how can i solve?
我正在编写一些关于 nlp 的代码。 我想训练并保存 model 但出现此错误。 我搜索了一些文档,但没有找到正确的解决方案。 我怎么解决这个问题?
import torch,time
import torch.nn as nn
input_dim = 5
hidden_dim = 10
n_layers = 1
lstm_layer = nn.LSTM(input_dim, hidden_dim, n_layers, batch_first=True)
batch_size = 1
seq_len = 1
inp = torch.randn(batch_size, seq_len, input_dim)
hidden_state = torch.randn(n_layers, batch_size, hidden_dim)
cell_state = torch.randn(n_layers, batch_size, hidden_dim)
hidden = (hidden_state, cell_state)
out, hidden = lstm_layer(inp, hidden)
print("Output shape: ", out.shape)
print("Hidden: ", hidden)
seq_len = 3
inp = torch.randn(batch_size, seq_len, input_dim)
out, hidden = lstm_layer(inp, hidden)
print(out.shape)
# Obtaining the last output
out = out.squeeze()[-1, :]
print(out.shape)
import bz2
from collections import Counter
import re
import nltk
import numpy as np
#nltk.download('punkt')
train_file = bz2.BZ2File('C:/Users/DELL/Dogal-Dil-Isleme/Xml-Files/trwiktionary-20200301-pages-articles-multistream.xml.bz2')
test_file = bz2.BZ2File('C:/Users/DELL/Dogal-Dil-Isleme/Xml-Files/trwikisource-20200601-pages-articles.xml.bz2')
train_file = train_file.readlines()
test_file = test_file.readlines()
num_train = 200
num_test = 50
train_file = [x.decode('utf-8') for x in train_file[:num_train]]
test_file = [x.decode('utf-8') for x in test_file[:num_test]]
train_labels = [0 if x.split(' ')[0] == '__label__1' else 1 for x in train_file]
train_sentences = [x.split(' ', 1)[1][:-1].lower() for x in train_file]
test_labels = [0 if x.split(' ')[0] == '__label__1' else 1 for x in test_file]
test_sentences = [x.split(' ', 1)[1][:-1].lower() for x in test_file]
for i in range(len(train_sentences)):
train_sentences[i] = re.sub('\d','0',train_sentences[i])
for i in range(len(test_sentences)):
test_sentences[i] = re.sub('\d','0',test_sentences[i])
for i in range(len(train_sentences)):
if 'www.' in train_sentences[i] or 'http:' in train_sentences[i] or 'https:' in train_sentences[i] or '.com' in train_sentences[i]:
train_sentences[i] = re.sub(r"([^ ]+(?<=\.[a-z]{3}))", "<url>", train_sentences[i])
for i in range(len(test_sentences)):
if 'www.' in test_sentences[i] or 'http:' in test_sentences[i] or 'https:' in test_sentences[i] or '.com' in test_sentences[i]:
test_sentences[i] = re.sub(r"([^ ]+(?<=\.[a-z]{3}))", "<url>", test_sentences[i])
words = Counter() # Dictionary that will map a word to the number of times it appeared in all the training sentences
for i, sentence in enumerate(train_sentences):
train_sentences[i] = []
for word in nltk.word_tokenize(sentence):
words.update([word.lower()])
train_sentences[i].append(word)
if i%20000 == 0:
print(str((i*100)/num_train) + "% done")
print("100% done")
words = {k:v for k,v in words.items() if v>1}
words = sorted(words, key=words.get, reverse=True)
words = ['_PAD','_UNK'] + words
word2idx = {o:i for i,o in enumerate(words)}
idx2word = {i:o for i,o in enumerate(words)}
for i, sentence in enumerate(train_sentences):
train_sentences[i] = [word2idx[word] if word in word2idx else 0 for word in sentence]
for i, sentence in enumerate(test_sentences):
# For test sentences, we have to tokenize the sentences as well
test_sentences[i] = [word2idx[word.lower()] if word.lower() in word2idx else 0 for word in nltk.word_tokenize(sentence)]
def pad_input(sentences, seq_len):
features = np.zeros((len(sentences), seq_len),dtype=int)
for ii, review in enumerate(sentences):
if len(review) != 0:
features[ii, -len(review):] = np.array(review)[:seq_len]
return features
seq_len = 200 # The length that the sentences will be padded/shortened to
train_sentences = pad_input(train_sentences, seq_len)
test_sentences = pad_input(test_sentences, seq_len)
train_labels = np.array(train_labels)
test_labels = np.array(test_labels)
split_frac = 0.5 # 50% validation, 50% test
split_id = int(split_frac * len(test_sentences))
val_sentences, test_sentences = test_sentences[:split_id], test_sentences[split_id:]
val_labels, test_labels = test_labels[:split_id], test_labels[split_id:]
import torch
from torch.utils.data import TensorDataset, DataLoader
import torch.nn as nn
train_data = TensorDataset(torch.from_numpy(train_sentences), torch.from_numpy(train_labels))
val_data = TensorDataset(torch.from_numpy(val_sentences), torch.from_numpy(val_labels))
test_data = TensorDataset(torch.from_numpy(test_sentences), torch.from_numpy(test_labels))
batch_size = 200
train_loader = DataLoader(train_data, shuffle=True, batch_size=batch_size)
val_loader = DataLoader(val_data, shuffle=True, batch_size=batch_size)
test_loader = DataLoader(test_data, shuffle=True, batch_size=batch_size)
# torch.cuda.is_available() checks and returns a Boolean True if a GPU is available, else it'll return False
is_cuda = torch.cuda.is_available()
# If we have a GPU available, we'll set our device to GPU. We'll use this device variable later in our code.
if is_cuda:
device = torch.device("cuda")
else:
device = torch.device("cpu")
class SentimentNet(nn.Module):
def __init__(self, vocab_size, output_size, embedding_dim, hidden_dim, n_layers, drop_prob=0.5):
super(SentimentNet, self).__init__()
self.output_size = output_size
self.n_layers = n_layers
self.hidden_dim = hidden_dim
self.embedding = nn.Embedding(vocab_size, embedding_dim)
self.lstm = nn.LSTM(embedding_dim, hidden_dim, n_layers, dropout=drop_prob, batch_first=True)
self.dropout = nn.Dropout(drop_prob)
self.fc = nn.Linear(hidden_dim, output_size)
self.sigmoid = nn.Sigmoid()
def forward(self, x, hidden):
batch_size = x.size(0)
x = x.long()
embeds = self.embedding(x)
lstm_out, hidden = self.lstm(embeds, hidden)
lstm_out = lstm_out.contiguous().view(-1, self.hidden_dim)
out = self.dropout(lstm_out)
out = self.fc(out)
out = self.sigmoid(out)
out = out.view(batch_size, -1)
out = out[:,-1]
return out, hidden
def init_hidden(self, batch_size):
weight = next(self.parameters()).data
hidden = (weight.new(self.n_layers, batch_size, self.hidden_dim).zero_().to(device),
weight.new(self.n_layers, batch_size, self.hidden_dim).zero_().to(device))
return hidden
vocab_size = len(word2idx) + 1
output_size = 1
embedding_dim = 400
hidden_dim = 512
n_layers = 2
model = SentimentNet(vocab_size, output_size, embedding_dim, hidden_dim, n_layers)
model.to(device)
lr=0.005
criterion = nn.BCELoss()
optimizer = torch.optim.Adam(model.parameters(), lr=lr)
epochs = 2
counter = 0
print_every = 1000
clip = 5
valid_loss_min = np.Inf
model.train()
for i in range(epochs):
h = model.init_hidden(batch_size)
for inputs, labels in train_loader:
counter += 1
h = tuple([e.data for e in h])
inputs, labels = inputs.to(device), labels.to(device)
model.zero_grad()
output, h = model(inputs, h)
loss = criterion(output.squeeze(), labels.float())
loss.backward()
nn.utils.clip_grad_norm_(model.parameters(), clip)
optimizer.step()
if counter%print_every == 0:
val_h = model.init_hidden(batch_size)
val_losses = []
model.eval()
for inp, lab in val_loader:
val_h = tuple([each.data for each in val_h])
inp, lab = inp.to(device), lab.to(device)
out, val_h = model(inp, val_h)
val_loss = criterion(out.squeeze(), lab.float())
val_losses.append(val_loss.item())
model.train()
print("Epoch: {}/{}...".format(i+1, epochs),
"Step: {}...".format(counter),
"Loss: {:.6f}...".format(loss.item()),
"Val Loss: {:.6f}".format(np.mean(val_losses)))
if np.mean(val_losses) <= valid_loss_min:
torch.save(model.state_dict(), 'C:/Users/DELL/Dogal-Dil-Isleme/Models/state_dict.pt')
print('Validation loss decreased ({:.6f} --> {:.6f}). Saving model ...'.format(valid_loss_min,np.mean(val_losses)))
valid_loss_min = np.mean(val_losses)
time.sleep(1)
# Loading the best model
model.load_state_dict(torch.load('C:/Users/DELL/Dogal-Dil-Isleme/Models/state_dict.pt'))
test_losses = []
num_correct = 0
h = model.init_hidden(batch_size)
model.eval()
for inputs, labels in test_loader:
h = tuple([each.data for each in h])
inputs, labels = inputs.to(device), labels.to(device)
output, h = model(inputs, h)
test_loss = criterion(output.squeeze(), labels.float())
test_losses.append(test_loss.item())
pred = torch.round(output.squeeze()) # Rounds the output to 0/1
correct_tensor = pred.eq(labels.float().view_as(pred))
correct = np.squeeze(correct_tensor.cpu().numpy())
num_correct += np.sum(correct)
print("Test loss: {:.3f}".format(np.mean(test_losses)))
test_acc = num_correct/len(test_loader.dataset)
print("Test accuracy: {:.3f}%".format(test_acc*100))
我尝试创建新文件夹并更改路径,但所有方法都出现错误:) 我阅读了 pytorch 文档并更改了推荐的代码,但错误仍然出现。 我将分享一些链接,供您阅读有关此问题的内容。
我该如何修复或有其他方法可以保存 model?
尝试将其更改为: model.load_state_dict(torch.load('C:/Users/DELL/Dogal-Dil-Isleme/Models/state_dict'))
声明:本站的技术帖子网页,遵循CC BY-SA 4.0协议,如果您需要转载,请注明本站网址或者原文地址。任何问题请咨询:yoyou2525@163.com.