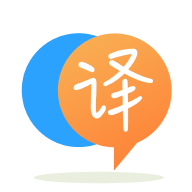
[英]Custom activation function in Tensorflow with trainable params
[英]How to return of one trainable parameters of custom activation function in PyTorch?
import torch
from another_file import Custom_activation_function as caf
class Example(torch.nn.Module):
def __init__(self):
super().__init__()
self.model = torch.nn.Sequential(
torch.nn.Linear(4, 250, bias=False),
caf(),
torch.nn.Linear(250, 250, bias=False),
caf(),
torch.nn.Linear(250, 1, bias=False),
)
def forward(self, x):
return model(x)
x = torch.randn((100, 4))
y = model(x)
下面提供了 Custom_activation_function 的伪代码:
class Custom_activation_function(torch.nn.Module):
def forward(self, x):
y = x * x
b = torch.exp(x)
return y
在上面的两段代码中,Custom_activation_function 是一个自定义激活 function 的 class,多个线性层和激活函数在一个顺序的 Z20F35E630DAF44DBFA4C3F68F5399D8Z 中捆绑在一起。 在训练 model 时,我们希望访问 Custom_activation_function 中定义的 b(例如,在损失 function 中的正则化项包括 b 的情况下)。 最好在不改变顺序 model 的情况下,在训练期间如何获得 b?
如果您需要访问b
作为中间 state (如在您的伪代码中),您可以尝试以下操作:
import torch
class caf(torch.nn.Module):
def __init__(self):
super().__init__()
def forward(self, x):
y = x * x
self.b = torch.exp(x)
return y
class Example(torch.nn.Module):
def __init__(self):
super().__init__()
self.model = torch.nn.Sequential(
torch.nn.Linear(4, 250, bias=False),
caf(),
torch.nn.Linear(250, 250, bias=False),
caf(),
torch.nn.Linear(250, 1, bias=False),
)
def forward(self, x):
return self.model(x)
my_model = Example()
optimizer = torch.optim.SGD(my_model.parameters(), lr=0.01)
loss_func = torch.nn.MSELoss()
x = torch.randn((100, 4))
y = torch.randn((100, 1))
for iteration in range(1000):
my_model.zero_grad()
out = my_model(x)
loss_base = loss_func(out, y)
loss_reg = []
# Identify your b's
for mod in my_model.model.modules():
if isinstance(mod, caf):
loss_reg.append(mod.b)
loss_reg = torch.stack(loss_reg).mean()
loss = loss_base + loss_reg
loss.backward()
optimizer.step()
print(loss.item(), loss_base.item(), loss_reg.item())
声明:本站的技术帖子网页,遵循CC BY-SA 4.0协议,如果您需要转载,请注明本站网址或者原文地址。任何问题请咨询:yoyou2525@163.com.