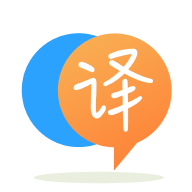
[英]Time Series Analysis - unevenly spaced measures - pandas + statsmodels
[英]time series analysis with statsmodels
我正在嘗試對時間序列數據進行多元回歸,但是當我將時間序列列添加到模型中時,最終將每個唯一值都視為一個單獨的變量,就像這樣(我的“日期”列的類型為datetime) :
est = smf.ols(formula='r ~ spend + date', data=df).fit()
print est.summary()
coef std err t P>|t| [95.0% Conf. Int.]
Intercept -6.249e-10 inf -0 nan nan nan
date[T.Timestamp('2014-10-08 00:00:00')] -2.571e-10 inf -0 nan nan nan
date[T.Timestamp('2014-10-15 00:00:00')] 9.441e-11 inf 0 nan nan nan
date[T.Timestamp('2014-10-22 00:00:00')] 5.619e-11 inf 0 nan nan nan
date[T.Timestamp('2014-10-29 00:00:00')] -8.035e-12 inf -0 nan nan nan
date[T.Timestamp('2014-11-05 00:00:00')] 6.334e-11 inf 0 nan nan nan
date[T.Timestamp('2014-11-12 00:00:00')] 7.9e+04 inf 0 nan nan nan
date[T.Timestamp('2014-11-19 00:00:00')] 1.58e+05 inf 0 nan nan nan
date[T.Timestamp('2014-11-26 00:00:00')] 1.58e+05 inf 0 nan nan nan
date[T.Timestamp('2014-12-03 00:00:00')] 1.58e+05 inf 0 nan nan nan
date[T.Timestamp('2014-12-10 00:00:00')] 2.28e+05 inf 0 nan nan nan
date[T.Timestamp('2014-12-17 00:00:00')] 3.28e+05 inf 0 nan nan nan
date[T.Timestamp('2014-12-24 00:00:00')] 3.705e+05 inf 0 nan nan nan
spend 2.105e-10 inf 0 nan nan nan
我還嘗試了statsmodel的tms包,但不確定如何處理“頻率”:
ar_model = sm.tsa.AR(df, freq='1')
ValueError: Pandas data cast to numpy dtype of object. Check input data with np.asarray(data).
我真的很想看看一個數據示例以及一個代碼片段,以重現您的錯誤。 否則,我的建議將無法解決您的特定錯誤消息。 但是,它將允許您對存儲在熊貓數據框中的一組時間序列進行多元回歸分析。 假設您在時間序列中使用的是連續值而不是分類值,這是我將如何使用pandas和statsmodels來做到這一點:
具有隨機值的數據框:
# Imports
import pandas as pd
import numpy as np
import itertools
np.random.seed(1)
rows = 12
listVars= ['y','x1', 'x2', 'x3']
rng = pd.date_range('1/1/2017', periods=rows, freq='D')
df_1 = pd.DataFrame(np.random.randint(100,150,size=(rows, len(listVars))), columns=listVars)
df_1 = df_1.set_index(rng)
print(df_1)
輸出-可以使用的一些數據:
y x1 x2 x3
2017-01-01 137 143 112 108
2017-01-02 109 111 105 115
2017-01-03 100 116 101 112
2017-01-04 107 145 106 125
2017-01-05 120 137 118 120
2017-01-06 111 142 128 129
2017-01-07 114 104 123 123
2017-01-08 141 149 130 132
2017-01-09 122 113 141 109
2017-01-10 107 122 101 100
2017-01-11 117 108 124 113
2017-01-12 147 142 108 130
下面的函數將讓您指定源數據幀以及因變量y和自變量x1,x2的選擇 。 使用statsmodels,一些期望的結果將存儲在數據框中。 在那里,R2將是數字類型的,而回歸系數和p值將是列表,因為這些估計的數量將隨您希望在分析中包括的自變量的數量而變化。
def LinReg(df, y, x, const):
betas = x.copy()
# Model with out without a constant
if const == True:
x = sm.add_constant(df[x])
model = sm.OLS(df[y], x).fit()
else:
model = sm.OLS(df[y], df[x]).fit()
# Estimates of R2 and p
res1 = {'Y': [y],
'R2': [format(model.rsquared, '.4f')],
'p': [model.pvalues.tolist()],
'start': [df.index[0]],
'stop': [df.index[-1]],
'obs' : [df.shape[0]],
'X': [betas]}
df_res1 = pd.DataFrame(data = res1)
# Regression Coefficients
theParams = model.params[0:]
coefs = theParams.to_frame()
df_coefs = pd.DataFrame(coefs.T)
xNames = list(df_coefs)
xValues = list(df_coefs.loc[0].values)
xValues2 = [ '%.2f' % elem for elem in xValues ]
res2 = {'Independent': [xNames],
'beta': [xValues2]}
df_res2 = pd.DataFrame(data = res2)
# All results
df_res = pd.concat([df_res1, df_res2], axis = 1)
df_res = df_res.T
df_res.columns = ['results']
return(df_res)
這是一個測試運行:
df_regression = LinReg(df = df, y = 'y', x = ['x1', 'x2'], const = True)
print(df_regression)
輸出:
results
R2 0.3650
X [x1, x2]
Y y
obs 12
p [0.7417691742514285, 0.07989515781898897, 0.25...
start 2017-01-01 00:00:00
stop 2017-01-12 00:00:00
Independent [const, x1, x2]
coefficients [16.29, 0.47, 0.37]
這是簡單復制粘貼的全部內容:
# Imports
import pandas as pd
import numpy as np
import statsmodels.api as sm
np.random.seed(1)
rows = 12
listVars= ['y','x1', 'x2', 'x3']
rng = pd.date_range('1/1/2017', periods=rows, freq='D')
df = pd.DataFrame(np.random.randint(100,150,size=(rows, len(listVars))), columns=listVars)
df = df.set_index(rng)
def LinReg(df, y, x, const):
betas = x.copy()
# Model with out without a constant
if const == True:
x = sm.add_constant(df[x])
model = sm.OLS(df[y], x).fit()
else:
model = sm.OLS(df[y], df[x]).fit()
# Estimates of R2 and p
res1 = {'Y': [y],
'R2': [format(model.rsquared, '.4f')],
'p': [model.pvalues.tolist()],
'start': [df.index[0]],
'stop': [df.index[-1]],
'obs' : [df.shape[0]],
'X': [betas]}
df_res1 = pd.DataFrame(data = res1)
# Regression Coefficients
theParams = model.params[0:]
coefs = theParams.to_frame()
df_coefs = pd.DataFrame(coefs.T)
xNames = list(df_coefs)
xValues = list(df_coefs.loc[0].values)
xValues2 = [ '%.2f' % elem for elem in xValues ]
res2 = {'Independent': [xNames],
'beta': [xValues2]}
df_res2 = pd.DataFrame(data = res2)
# All results
df_res = pd.concat([df_res1, df_res2], axis = 1)
df_res = df_res.T
df_res.columns = ['results']
return(df_res)
df_regression = LinReg(df = df, y = 'y', x = ['x1', 'x2'], const = True)
print(df_regression)
您可以為每個日期擬合一個線性模型,因為ols將日期視為分類變量。 我建議您嘗試:
est = smf.ols(formula='r ~ spend', data=df).fit()
print est.summary()
對於statsmodel,請嘗試:
ar_model = sm.tsa.AR(df['spend'], freq='1')
聲明:本站的技術帖子網頁,遵循CC BY-SA 4.0協議,如果您需要轉載,請注明本站網址或者原文地址。任何問題請咨詢:yoyou2525@163.com.