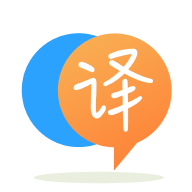
[英]I am trying to build a neural network with one neuron using the pytorch library. It keeps giving me an error
[英]Giving output of one neural network as an input to another in pytorch
我有一個預訓練的卷積神經網絡,它產生和輸出形狀 (X,164),其中 X 是測試示例的數量。 所以輸出層有 164 個節點。 我想獲取這個輸出並給這兩個網絡提供另一個網絡,它只是一個完全連接的神經網絡,其中第一層有 64 個節點,輸出層有 1 個具有 sigmoid 函數的節點。 我怎樣才能做到這一點? 我的第一個網絡看起來像:
class LambdaBase(nn.Sequential):
def __init__(self, fn, *args):
super(LambdaBase, self).__init__(*args)
self.lambda_func = fn
def forward_prepare(self, input):
output = []
for module in self._modules.values():
output.append(module(input))
return output if output else input
class Lambda(LambdaBase):
def forward(self, input):
return self.lambda_func(self.forward_prepare(input))
class LambdaMap(LambdaBase):
def forward(self, input):
return list(map(self.lambda_func,self.forward_prepare(input)))
class LambdaReduce(LambdaBase):
def forward(self, input):
return reduce(self.lambda_func,self.forward_prepare(input))
def get_model(load_weights = True):
pretrained_model_reloaded_th = nn.Sequential( # Sequential,
nn.Conv2d(4,300,(19, 1)),
nn.BatchNorm2d(300),
nn.ReLU(),
nn.MaxPool2d((3, 1),(3, 1)),
nn.Conv2d(300,200,(11, 1)),
nn.BatchNorm2d(200),
nn.ReLU(),
nn.MaxPool2d((4, 1),(4, 1)),
nn.Conv2d(200,200,(7, 1)),
nn.BatchNorm2d(200),
nn.ReLU(),
nn.MaxPool2d((4, 1),(4, 1)),
Lambda(lambda x: x.view(x.size(0),-1)), # Reshape,
nn.Sequential(Lambda(lambda x: x.view(1,-1) if 1==len(x.size()) else x ),nn.Linear(2000,1000)), # Linear,
nn.BatchNorm1d(1000,1e-05,0.1,True),#BatchNorm1d,
nn.ReLU(),
nn.Dropout(0.3),
nn.Sequential(Lambda(lambda x: x.view(1,-1) if 1==len(x.size()) else x ),nn.Linear(1000,1000)), # Linear,
nn.BatchNorm1d(1000,1e-05,0.1,True),#BatchNorm1d,
nn.ReLU(),
nn.Dropout(0.3),
nn.Sequential(Lambda(lambda x: x.view(1,-1) if 1==len(x.size()) else x ),nn.Linear(1000,164)), # Linear,
nn.Sigmoid(),
)
if load_weights:
sd = torch.load('pretrained_model.pth')
pretrained_model_reloaded_th.load_state_dict(sd)
return pretrained_model_reloaded_th
model = get_model(load_weights = True)
如果我想在我的測試集上獲得這個模型的輸出,我可以簡單地做:
output = model(X.float())
這將產生形狀 (X,164) 的最終輸出。 現在我想把這個輸出交給上面提到的另一個神經網絡。 我現在如何將這兩個網絡結合起來,如何一起優化這些網絡? 見解將不勝感激。
編輯:我的第二個模型是:
# define second model architecture
next_model = nn.Sequential(
nn.Linear(164, 64),
nn.ReLU(),
nn.Linear(64, 1),
nn.Sigmoid()
)
# print model architecture
print(next_model)
我的分類器被訓練為:
for epoch in range(2): # loop over the dataset multiple times
running_loss = 0.0
for i, data in enumerate(trainloader, 0):
# get the inputs; data is a list of [inputs, labels]
inputs, labels = data
# zero the parameter gradients
optimizer.zero_grad()
# forward + backward + optimize
outputs = model(inputs)
loss = criterion(outputs, labels)
loss.backward()
optimizer.step()
# print statistics
running_loss += loss.item()
if i % 2000 == 1999: # print every 2000 mini-batches
print('[%d, %5d] loss: %.3f' %
(epoch + 1, i + 1, running_loss / 2000))
running_loss = 0.0
print('Finished Training')
如果這兩個模型不需要在第一個模型輸出時進行任何調整,您可以簡單地使用nn.Sequential
:
>>> network = nn.Sequential(model, next_model)
並以與使用model
相同的方式使用它:
>>> output = network(X.float())
這將對應於next_model(model(X.float()))
。
聲明:本站的技術帖子網頁,遵循CC BY-SA 4.0協議,如果您需要轉載,請注明本站網址或者原文地址。任何問題請咨詢:yoyou2525@163.com.