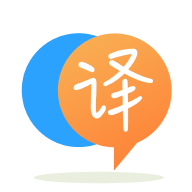
[英]Expected convolution2d_input_1 to have 4 dimensions, but got array with shape error in keras
[英]Keras Convolution2D Input: Error when checking model input: expected convolution2d_input_1 to have shape
我正在完成这个使用Keras创建图像分类器的精彩教程 。 一旦我训练了模型,我将其保存到一个文件中,然后将其重新加载到下面显示的测试脚本中的模型中。
当我使用新的,前所未见的图像评估模型时,我得到以下异常:
错误:
Traceback (most recent call last):
File "test_classifier.py", line 48, in <module>
score = model.evaluate(x, y, batch_size=16)
File "/Library/Python/2.7/site-packages/keras/models.py", line 655, in evaluate
sample_weight=sample_weight)
File "/Library/Python/2.7/site-packages/keras/engine/training.py", line 1131, in evaluate
batch_size=batch_size)
File "/Library/Python/2.7/site-packages/keras/engine/training.py", line 959, in _standardize_user_data
exception_prefix='model input')
File "/Library/Python/2.7/site-packages/keras/engine/training.py", line 108, in standardize_input_data
str(array.shape))
Exception: Error when checking model input: expected convolution2d_input_1 to have shape (None, 3, 150, 150) but got array with shape (1, 3, 150, 198)`
是我训练的模型的问题还是我如何调用evaluate方法?
码:
from keras.preprocessing.image import ImageDataGenerator
from keras.models import Sequential
from keras.layers import Convolution2D, MaxPooling2D
from keras.layers import Activation, Dropout, Flatten, Dense
from keras.preprocessing.image import ImageDataGenerator, array_to_img, img_to_array, load_img
import numpy as np
img_width, img_height = 150, 150
train_data_dir = 'data/train'
validation_data_dir = 'data/validation'
nb_train_samples = 2000
nb_validation_samples = 800
nb_epoch = 5
model = Sequential()
model.add(Convolution2D(32, 3, 3, input_shape=(3, img_width, img_height)))
model.add(Activation('relu'))
model.add(MaxPooling2D(pool_size=(2, 2)))
model.add(Convolution2D(32, 3, 3))
model.add(Activation('relu'))
model.add(MaxPooling2D(pool_size=(2, 2)))
model.add(Convolution2D(64, 3, 3))
model.add(Activation('relu'))
model.add(MaxPooling2D(pool_size=(2, 2)))
model.add(Flatten())
model.add(Dense(64))
model.add(Activation('relu'))
model.add(Dropout(0.5))
model.add(Dense(1))
model.add(Activation('sigmoid'))
model.compile(loss='binary_crossentropy',
optimizer='rmsprop',
metrics=['accuracy'])
model.load_weights('first_try.h5')
img = load_img('data/test2/ferrari.jpeg')
x = img_to_array(img) # this is a Numpy array with shape (3, 150, 150)
x = x.reshape( (1,) + x.shape ) # this is a Numpy array with shape (1, 3, 150, 150)
y = np.array([0])
score = model.evaluate(x, y, batch_size=16)`
问题是由于测试图像的大小错误。 为了我,
train_datagen.flow_from_directory(
'C:\\Users\\...\\train', # this is the target directory
target_size=(150, 150), # all images will be resized to 150x150
batch_size=32,
class_mode='binary')
工作不正常。 所以我使用matlab
命令调整所有测试图像的大小,它工作正常
这个问题有两个方面:
测试图像尺寸错误。 它是150 x 198,需要150 x 150。
我不得不将密集层从model.add(Dense(10))
更改为model.add(Dense(1))
。
我还不知道如何让模型给我预测,但至少现在,模型评估运行。
我有同样的问题并使用此功能:目标文件夹(.jpg和.png)中的所有图像将调整为高度和宽度。 并除以255.另外增加了1个维度(所需的输入形状)。
from scipy import misc
import os
def readImagesAsNumpyArrays(targetPath, i_height, i_width):
files = os.listdir(targetPath)
npList = list()
for file in files:
if ".jpg" or ".png" in str(file):
path = os.path.join(targetPath, file)
img = misc.imread(path)
img = misc.imresize(img, (i_height, i_width))
img = img * (1. / 255)
img = img[None, :, :,: ]
npList.append(img)
return npList
声明:本站的技术帖子网页,遵循CC BY-SA 4.0协议,如果您需要转载,请注明本站网址或者原文地址。任何问题请咨询:yoyou2525@163.com.