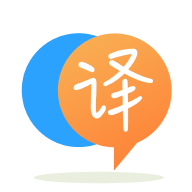
[英]ValueError: Error when checking input: expected embedding_1_input to have shape (256,) but got array with shape (1,)
[英]Error when checking input: expected embedding_1_input to have shape (50,) but got array with shape (1,)
我有一个错误:
Error when checking input:
expected embedding_1_input to have shape (50,) but got array with shape (1,)
当我将输入参数input_length
更改为 1 时,错误变为:
Error when checking input:
expected embedding_1_input to have shape (1,) but got array with shape (50,)
我的代码如下:
from sklearn.feature_extraction.text import CountVectorizer, TfidfTransformer, TfidfVectorizer
import numpy as np
import os
from keras import metrics
from keras.layers import Dense, Dropout, Embedding, LSTM, Bidirectional, BatchNormalization, Activation, Conv1D, MaxPooling1D, Flatten, GlobalMaxPooling1D
from keras.models import load_model
from keras.callbacks import ModelCheckpoint
from sklearn.preprocessing import LabelEncoder
from sklearn.model_selection import train_test_split
from keras.models import Sequential
from keras.preprocessing import sequence, text
import pandas as pd
from gensim import corpora
from gensim import models
maxlen = 50
batch_size = 128
np.random.seed(7)
df = pd.read_csv('C:/Users/DMY/Peer-logic-master/newdata/topnine.csv',encoding='utf-8')
x = df["REVIEW"].fillna("na").values
y = df["TAG"]
encoder = LabelEncoder()
encoder.fit(y)
y = encoder.transform(y)
x_train,x_test,y_train,y_test = train_test_split(x,y,test_size=0.1)
word_list = []
for i in range(len(x_train)):
word_list.append(x_train[i].split(' '))
dictionary = corpora.Dictionary(word_list)
corpus = [dictionary.doc2bow(text) for text in word_list]
tfidf = models.TfidfModel(corpus)
X_train_id = []
word_id_dict = dictionary.token2id
for i in range(len(word_list)):
sen_id = []
word_sen = word_list[i]
for j in range(len(word_sen)):
id = word_id_dict.get(word_sen[j])
if id is None:
id = 0
sen_id.append(id)
X_train_id.append(sen_id)
X_train_tfidf_vec = []
for i in range(len(x_train)):
temp = {}
string = x_train[i]
string_bow = dictionary.doc2bow(string.lower().split())
string_tfidf = tfidf[string_bow]
for j in range(len(string_tfidf)):
# print(string_tfidf[j][0])
temp[string_tfidf[j][0]] = string_tfidf[j][1]
# print(temp)
X_train_tfidf_vec.append(temp)
X_train_tfidf = []
for i in range(len(X_train_id)):
sen_id = X_train_id[i]
sen_id_tfidf = X_train_tfidf_vec[i]
sen = []
for j in range(len(sen_id)):
word_id = sen_id[j]
word_tfidf = sen_id_tfidf.get(word_id)
if word_tfidf is None:
word_tfidf = 0
sen.append(word_tfidf)
X_train_tfidf.append(sen)
x_train_tfidf = sequence.pad_sequences(X_train_tfidf, maxlen=maxlen,dtype='float64')
#print(len(x_train_tfidf))
#print(x_train_tfidf)
model4 = Sequential()
model4.add(Embedding(len(x_train_tfidf)+1, 100, input_length = ))#input_dim,output_dim,input_length
model4.add(Dropout(0.6))
model4.add(LSTM(100, recurrent_dropout=0.6))
model4.add(Dropout(0.6))
model4.add(Dense(1, activation='sigmoid'))
model4.compile('adam', 'binary_crossentropy', metrics=['accuracy'])
model4_history = model4.fit(x_train_tfidf, y_train, batch_size=batch_size, epochs=7,
validation_split=0.1)
score4, acc4 = model4.evaluate(x_test, y_test,
batch_size=batch_size)
print('Test accuracy for LSTM Model is:', acc4)
y_pred4 = model4.predict(x_test)
y_pred4 = (y_pred4 > 0.5)
print(classification_report(y_test, y_pred4))
根据官方文档,嵌入层采用了许多不同的初始化参数。
Embedding(input_dim,
output_dim,
embeddings_initializer='uniform',
embeddings_regularizer=None,
activity_regularizer=None,
embeddings_constraint=None,
mask_zero=False,
input_length=None)
注意input_dim
指定了输入数据的词汇量大小,即数据中有多少个不同的词。 output_dim
指定了潜在空间的维度,即嵌入变换后每个词的向量的长度。
让我们通过一个例子来理解这一点。 考虑以下层:
model.add(Embedding(1000, 64, input_length=10))
这说明了这样一个事实
该层的输出形状为(None, 10, 64)
,其中None
是批量大小。 在您的情况下, input_length
可能应该设置为等于maxlen
,这似乎指定了输入数据中每个实例的大小。
声明:本站的技术帖子网页,遵循CC BY-SA 4.0协议,如果您需要转载,请注明本站网址或者原文地址。任何问题请咨询:yoyou2525@163.com.