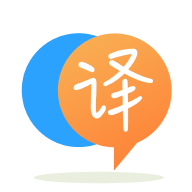
[英]Multi-class for sentence classification with pytorch (Using nn.LSTM)
[英]pytorch multi-class lstm predicting all one class on testing
我正在做一个项目(我的第一个 AI 项目),但遇到了一些障碍。 在我训练的分类器上执行测试时,它预测一切都属于第 1 类。现在数据集严重偏向第 1 类; 但是,我已经实施了权重来弥补这一点。 只是担心我编码错误或遗漏了什么。 如果你看到任何东西,请告诉我。
这是设置和培训
batchSize = 50
trainingLoad = DataLoader(trainingData, shuffle = True, batch_size = batchSize, drop_last=True)
validationLoad = DataLoader(validationData, shuffle = True, batch_size = batchSize, drop_last=True)
testingLoad = DataLoader(testingData, shuffle = True, batch_size = batchSize, drop_last=True)
vocabularySize = len(wordToNoDict)
output = 3
embedding = 400
hiddenDimension = 524
layers = 4
classifierModel = Classifier.HateSpeechDetector(device, vocabularySize, output, embedding, hiddenDimension, layers)
classifierModel.to(device)
path = 'Program\data\state_dict2.pt'
weights = torch.tensor([1203/1203, 1203/15389, 1203/3407])
criterion = nn.CrossEntropyLoss(weight = weights)
trainClassifier(classifierModel, trainingLoad, validationLoad, device, batchSize, criterion, path)
test(classifierModel, path, testingLoad, batchSize, device, criterion)
def trainClassifier(model, trainingData, validationData, device, batchSize, criterion, path):
epochs = 5
counter = 0
testWithValiEvery = 10
clip = 5
valid_loss_min = np.Inf
lr=0.0001
optimizer = torch.optim.Adam(model.parameters(), lr=lr)
model.train()
for i in range(epochs):
h = model.init_hidden(batchSize, device)
for inputs, labels in trainingData:
h = tuple([e.data for e in h])
inputs, labels = inputs.to(device), labels.to(device)
model.zero_grad()
output, h = model(inputs, h)
loss = criterion(output.squeeze(), labels.long())
loss.backward()
nn.utils.clip_grad_norm_(model.parameters(), clip)
optimizer.step()
counter += 1
print(counter)
if counter%testWithValiEvery == 0:
print("validating")
val_h = model.init_hidden(batchSize, device)
val_losses = []
model.eval()
for inp, lab in validationData:
val_h = tuple([each.data for each in val_h])
inp, lab = inp.to(device), lab.to(device)
out, val_h = model(inp, val_h)#
val_loss = criterion(out.squeeze(), lab.long())
val_losses.append(val_loss.item())
model.train()
print("Epoch: {}/{}...".format(i+1, epochs),
"Step: {}...".format(counter),
"Loss: {:.6f}...".format(loss.item()),
"Val Loss: {:.6f}".format(np.mean(val_losses)))
if np.mean(val_losses) <= valid_loss_min:
torch.save(model.state_dict(), path)
print('Validation loss decreased ({:.6f} --> {:.6f}). Saving model ...'.format(valid_loss_min,np.mean(val_losses)))
print('model saved')
valid_loss_min = np.mean(val_losses)
这是分类器 - 这里有相当数量的随机评论,我已经干预了比特
import torch
import torch.nn as nn
import torch.nn.functional as F
import torch.optim as op
import torchvision
from torch.utils.data import TensorDataset, DataLoader
from torchvision import transforms, datasets
class HateSpeechDetector(nn.Module):
def __init__(self, device, vocabularySize, output, embedding, hidden, layers, dropProb=0.5):
super(HateSpeechDetector, self).__init__()
#Number of outputs (Classes/Categories)
self.output = output
#Number of layers in the LSTM
self.numLayers = layers
#Number of hidden neurons in each LSTM layer
self.hiddenDimensions = hidden
#Device being used for by model (CPU or GPU)
self.device = device
#Embedding layer finds correlations in words by converting word integers into vectors
self.embedding = nn.Embedding(vocabularySize, embedding)
#LSTM stores important data in memory, using it to help with future predictions
self.lstm = nn.LSTM(embedding,hidden,layers,dropout=dropProb,batch_first=True)
#Dropout is used to randomly drop nodes. This helps to prevent overfitting of the model during training
self.dropout = nn.Dropout(dropProb)
#Establishing 4 simple layers and a sigmoid output
self.fc = nn.Linear(hidden, hidden)
self.fc2 = nn.Linear(hidden, hidden)
self.fc3 = nn.Linear(hidden, hidden)
self.fc4 = nn.Linear(hidden, hidden)
self.fc5 = nn.Linear(hidden, hidden)
self.fc6 = nn.Linear(hidden, output)
self.softmax = nn.Softmax(dim=2)
def forward(self, x, hidden):
batchSize = x.size(0)
x = x.long()
embeds = self.embedding(x)
lstm_out, hidden = self.lstm(embeds, hidden)
#Tensor changes here from 250,33,524 to 8250,524
# lstm_out = lstm_out.contiguous().view(-1,self.hiddenDimensions)
out = self.dropout(lstm_out)
out = self.fc(out)
out = self.fc2(out)
out = self.fc3(out)
out = self.fc4(out)
out = self.fc5(out)
out = self.fc6(out)
out = self.softmax(out)
out = out[:,-1,:]
# myTensor = torch.Tensor([0,0,0])
# newOut = torch.zeros(batchSize, self.output)
# count = 0
# row = 0
# for tensor in out:
# if(count == 33):
# newOut[row] = myTensor/33
# myTensor = torch.Tensor([0,0,0])
# row += 1
# count = 0
# myTensor += tensor
# count += 1
return out, hidden
def init_hidden(self, batchSize, device):
weight = next(self.parameters()).data
hidden = (weight.new(self.numLayers, batchSize, self.hiddenDimensions).zero_().to(device), weight.new(self.numLayers, batchSize, self.hiddenDimensions).zero_().to(device))
return hidden
您已经为交叉熵损失添加了权重,并且权重已经偏向第一类 ( [1.0, 0.08, 0.35]
)。
对某个类具有更高的权重意味着模型将因该类错误而受到更严重的惩罚,并且模型有可能学习仅将所有内容预测为具有最高权重的类。 通常您不需要手动分配权重。
此外,检查您的数据以查看是否存在标签不平衡,即您是否有更多的第一类训练示例。 不平衡的训练集与为损失设置不同的权重具有相似的效果。
声明:本站的技术帖子网页,遵循CC BY-SA 4.0协议,如果您需要转载,请注明本站网址或者原文地址。任何问题请咨询:yoyou2525@163.com.